Primary Hero
description text

description text
Microsite Carousel - Padding Both
Microsite Carousel - Padding Both
Optional Title - bottom padding
[Optional] Ut enim ad minim veniam, quis nostrud exercitation ullamco laboris nisi ut aliquip ex ea commodo consequat. Duis aute irure dolor in reprehenderit in voluptate velit esse cillum dolore eu fugiat nulla pariatur.
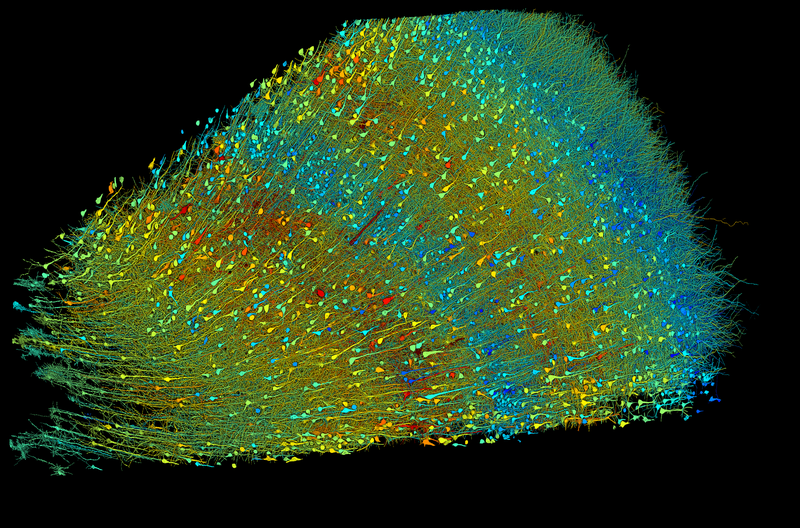
Case Studye
Title here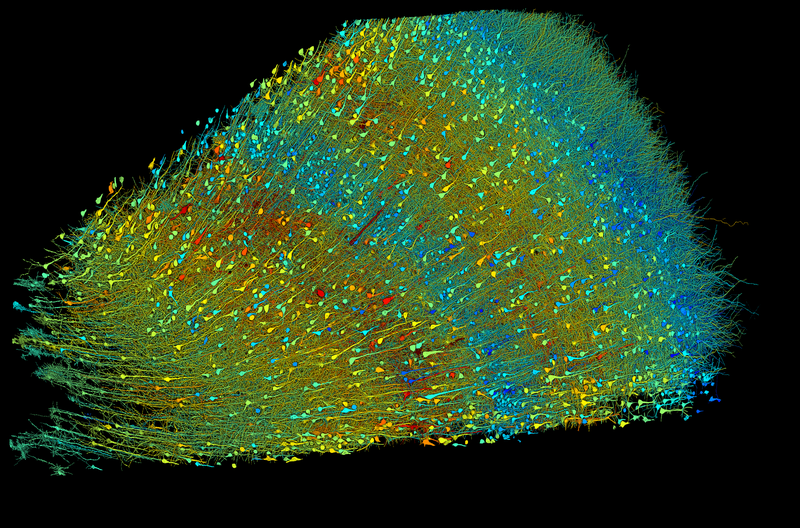
Case Studye
Title here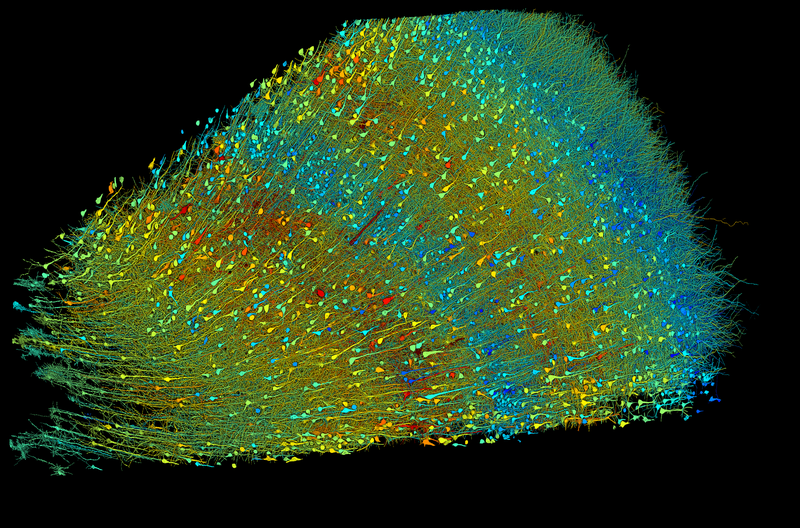
Case Studye
Title here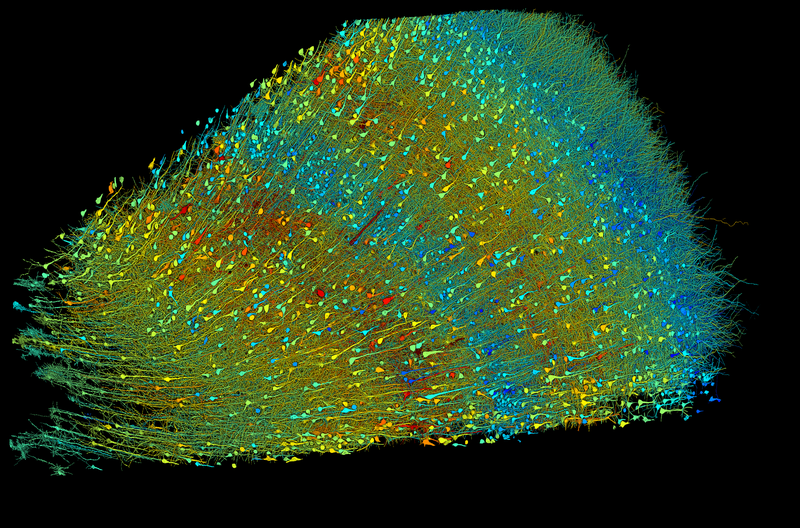
Case Studye
Title hereOptional Title - bottom padding
[Optional] Ut enim ad minim veniam, quis nostrud exercitation ullamco laboris nisi ut aliquip ex ea commodo consequat. Duis aute irure dolor in reprehenderit in voluptate velit esse cillum dolore eu fugiat nulla pariatur.
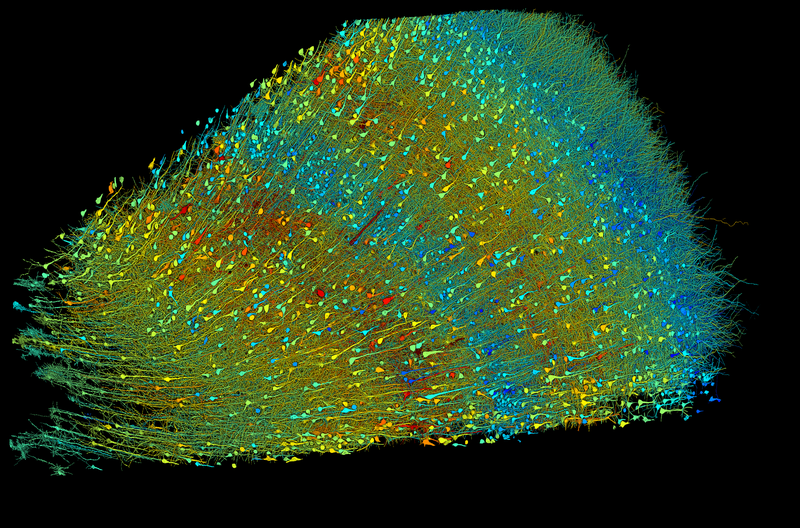
Case Studye
Title here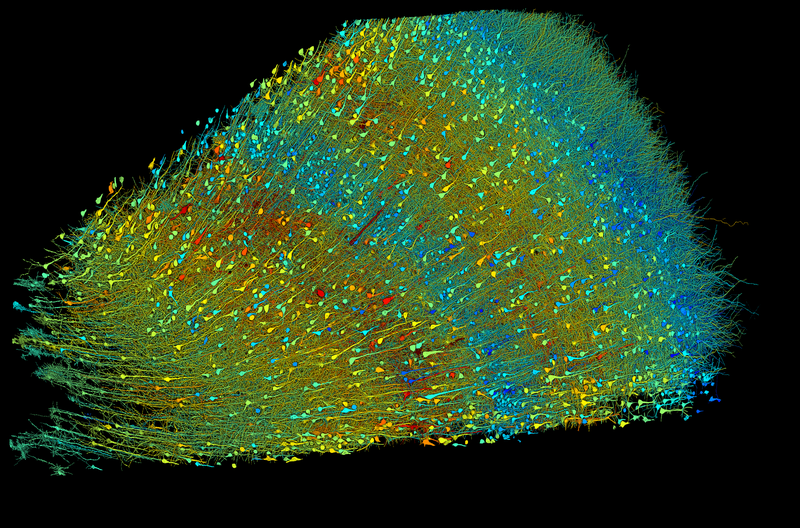
Case Studye
Title here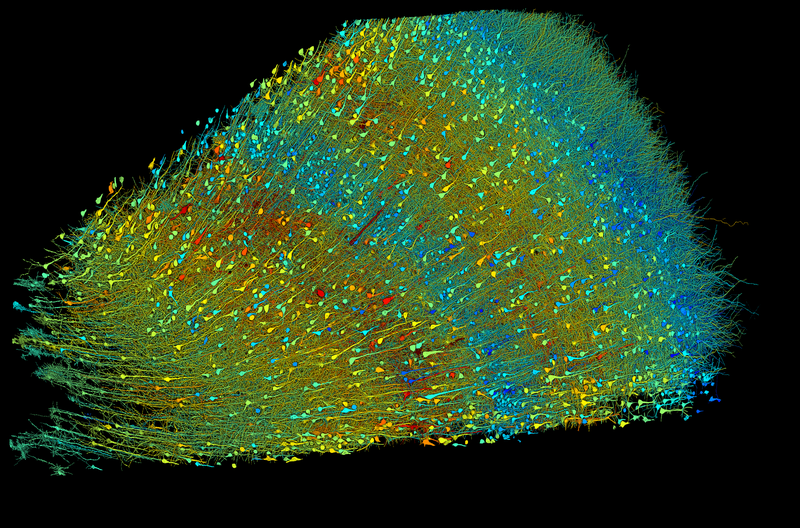
Case Studye
Title here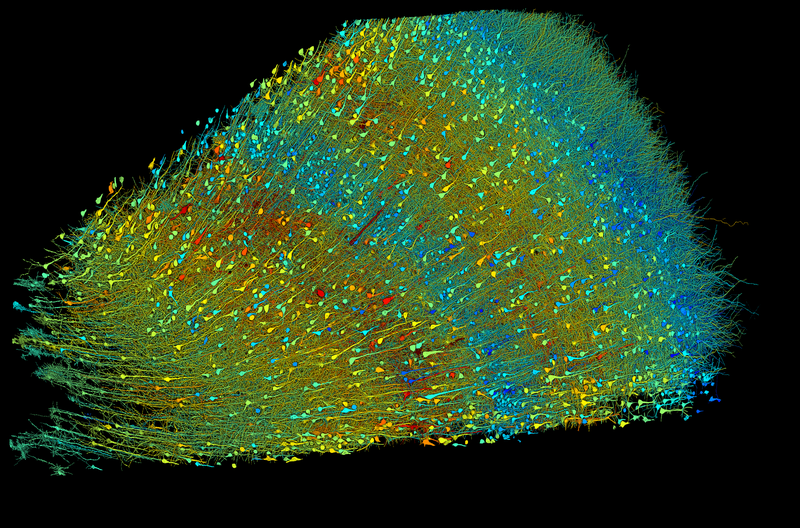
Case Studye
Title hereAcknowledgements[cc4ac8]
We give special thanks to the Imagen Video team for their collaboration and for providing their system to do super resolution. To our artist friends Irina Blok and Alonso Martinez for extensive creative exploration of the system and for using Phenaki to generate some of the videos showcased here. We also want to thank Niki Parmar for initial discussions. Special thanks to Gabriel Bender and Thang Luong for reviewing the paper and providing constructive feedback. We appreciate the efforts of Kevin Murphy and David Fleet for advising the project and providing feedback throughout. We are grateful to Evan Rapoport, Douglas Eck and Zoubin Ghahramani for supporting this work in a variety of ways. Tim Salimans and Chitwan Saharia helped us with brainstorming and coming up with shared benchmarks. Jason Baldridge was instrumental for bouncing ideas. Alex Rizkowsky was very helpful in keeping things organized, while Erica Moreira and Victor Gomes ensured smooth resourcing for the project. Sarah Laszlo and Kathy Meier-Hellstern have greatly helped us incorporate important responsible AI practices into this project, which we are immensely grateful for. Finally, Blake Hechtman and Anselm Levskaya were generous in helping us debug a number of JAX issues.
Credit for Phenakistoscope asset:
Creator: Muybridge, Eadweard, 1830-1904, artist
Title: The zoopraxiscope* - a couple waltzing (No. 35., title from item.)
Edits made: Extended background and converted file format to mp4
Acknowledgements[cc4ac8]
We give special thanks to the Imagen Video team for their collaboration and for providing their system to do super resolution. To our artist friends Irina Blok and Alonso Martinez for extensive creative exploration of the system and for using Phenaki to generate some of the videos showcased here. We also want to thank Niki Parmar for initial discussions. Special thanks to Gabriel Bender and Thang Luong for reviewing the paper and providing constructive feedback. We appreciate the efforts of Kevin Murphy and David Fleet for advising the project and providing feedback throughout. We are grateful to Evan Rapoport, Douglas Eck and Zoubin Ghahramani for supporting this work in a variety of ways. Tim Salimans and Chitwan Saharia helped us with brainstorming and coming up with shared benchmarks. Jason Baldridge was instrumental for bouncing ideas. Alex Rizkowsky was very helpful in keeping things organized, while Erica Moreira and Victor Gomes ensured smooth resourcing for the project. Sarah Laszlo and Kathy Meier-Hellstern have greatly helped us incorporate important responsible AI practices into this project, which we are immensely grateful for. Finally, Blake Hechtman and Anselm Levskaya were generous in helping us debug a number of JAX issues.
Credit for Phenakistoscope asset:
Creator: Muybridge, Eadweard, 1830-1904, artist
Title: The zoopraxiscope* - a couple waltzing (No. 35., title from item.)
Edits made: Extended background and converted file format to mp4
Microsite Carousel - Padding Both
Microsite Carousel - Padding Both
Code block - Padding Bottom
this is the caption
Dynamic Accordion - Padding Bottom
asdfasdfasdfasdf
asdfasdfasdfasdf
asdfasdfasdfasdf
Dynamic Accordion - Padding Both
asdfasdfasdfasdf
asdfasdfasdfasdf
asdfasdfasdfasdf
Dynamic Basic Card Stack - Padding Bottom
Dynamic Basic Card Stack - Padding Both
Multi-Column - Both Padding
-
Top Caption
-
Top Caption
-
Top Caption
-
Top Caption
Multi-Column - Both Padding
-
Top Caption
-
Top Caption
-
Top Caption
-
Top Caption
Mixed Publications List - Bottom Padding
Mixed Publications List - Bottom Padding
Quote - Padding both
quote
Quote - Padding Bottom
quote