Researcher Spotlight
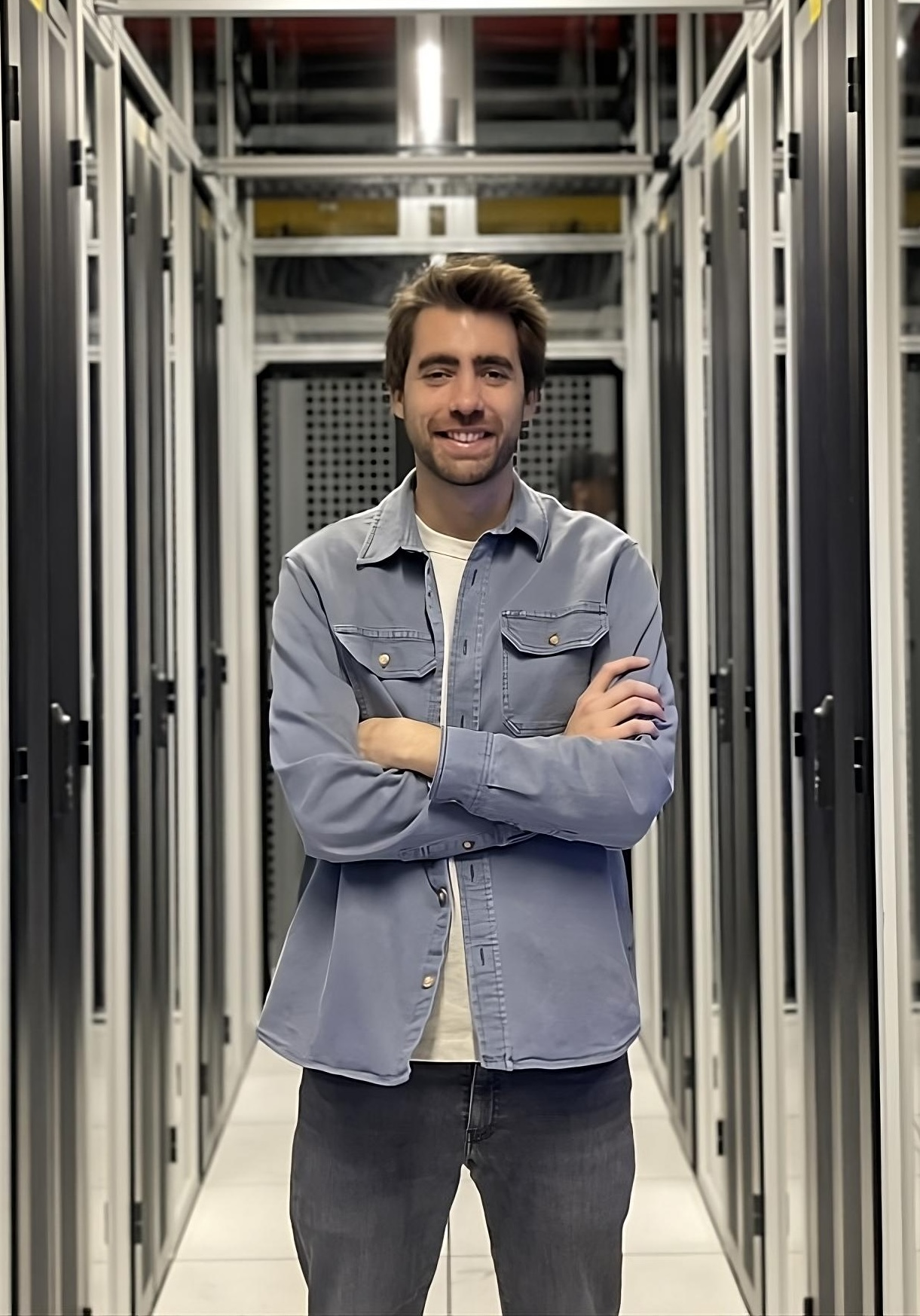
Alexandre Laterre
Hi, I'm Alex! I lead the Research & Development team at InstaDeep, a UK-based company specialising in Artificial Intelligence for Life Sciences and Industrial Optimisation. Together with my team, we conduct fundamental research in Machine Learning and explore its early applications to real-world problems.
In 2022, we joined the TPU Research Cloud (TRC) program, which quickly became our primary infrastructure for all our research. TRC's support has enabled us to scale experiments, take on ambitious projects, and achieve high-impact scientific results.
In Life Sciences, we developed state-of-the-art foundation models for plants (Nature Communications Biology, Google Cloud blog) and human genomics (Nature Methods). We also pioneered the use of Bayesian Flow Networks — a new class of generative models — in digital biology. Our latest models, ProtBFN and AbBFN (preprint) set a new benchmark in amino acid sequence generation (e.g. proteins and antibodies), supporting the design of new therapeutics.
In Reinforcement Learning (RL), TRC supported our contribution to open-source, including several impactful RL projects: Jumanji (high-performance RL environments in JAX), Flashbax (replay buffer), Mava (framework for multi-agent RL), and Sebulba (optimised training pipeline for real-world RL applications, Google Cloud blog).
TRC has been vital to our progress, empowering us to innovate and share our work globally. I'm deeply grateful for their continued support.
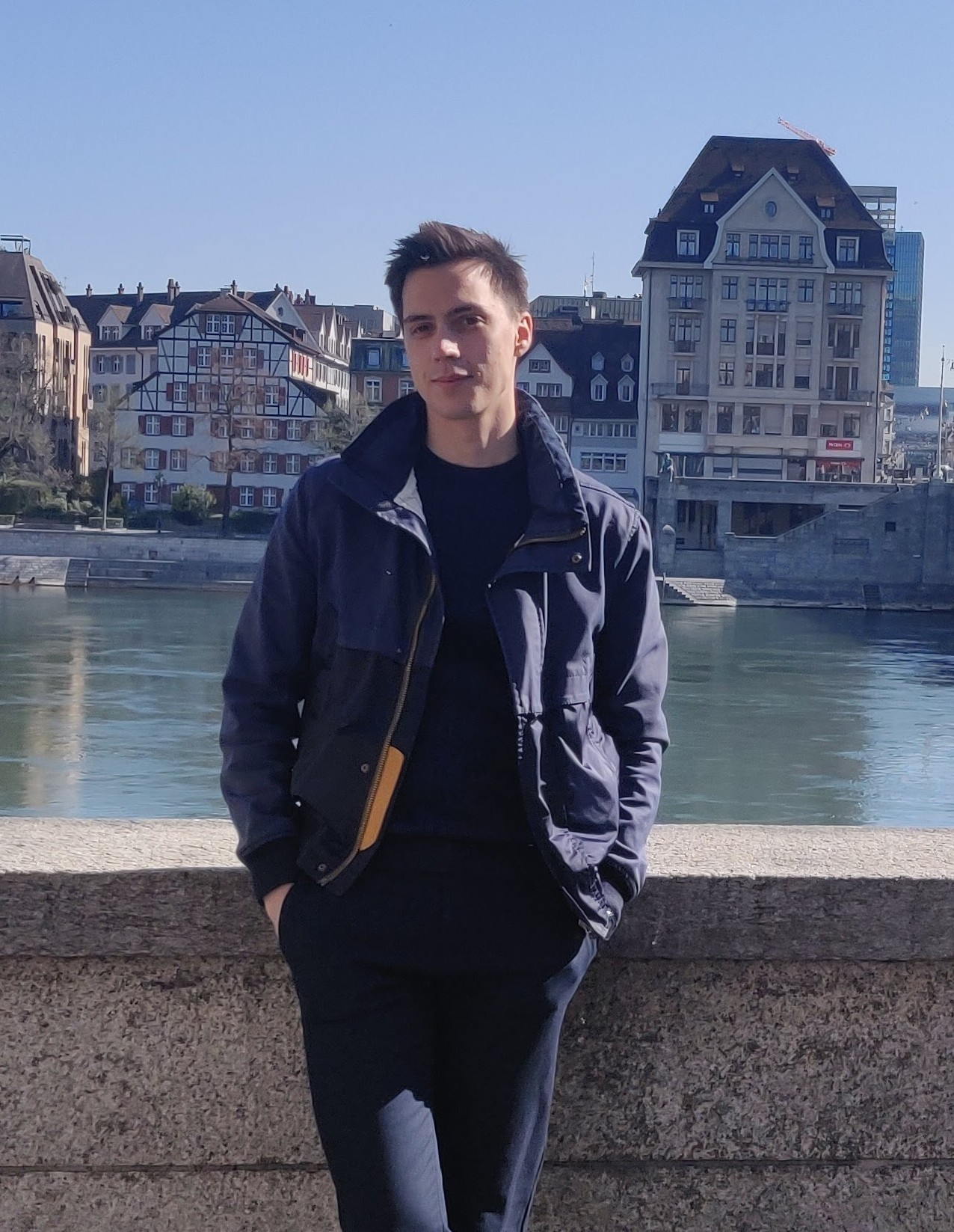
Dan Graur
Hi! My name is Dan Graur, I'm a PhD student in the Systems Group at ETH Zurich. I work on Systems for Machine Learning together with Prof. Ana Klimovic, specifically on trying to improve the performance of ML workloads by eliminating preprocessing bottlenecks which stall training. The support from TRC has been instrumental to our work.
We built Cachew, an open-source Preprocessing-as-a-Service framework based on TensorFlow and tf.data. Cachew introduces policies for (1) autoscaling data preprocessing resources to avoid data stalls during training and (2) caching preprocessed data to maximize training throughput. These policies work transparently, without requiring practitioners to make challenging resource allocation and data management decisions in response to real-time changes in their ML deployments. TRC has allowed us to experiment with many policies and deploy ML models on high-performance TPUs which push preprocessing to its limits, the ideal scenario for our use-case. We are currently looking into other ways of improving ML training efficiency with continued support from TRC.
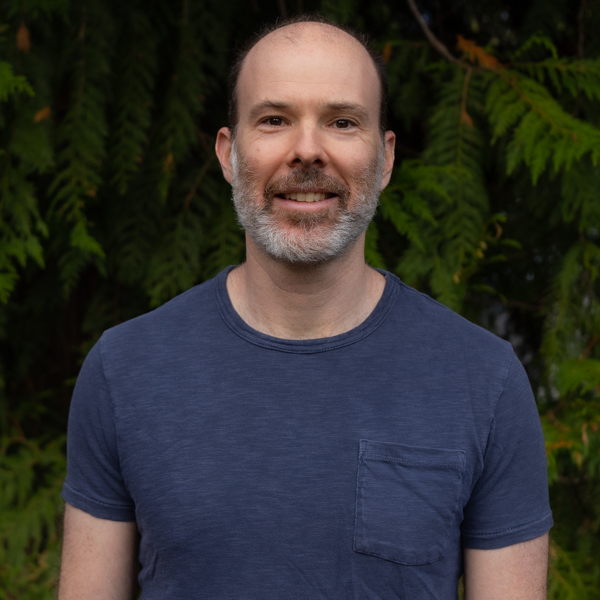
Ross Wightman
Hello, I'm Ross Wightman, creator of the PyTorch Image Models (timm) open-source library. With almost 800 pretrained weights, and 1000 architecture variations, timm is the largest collection of image classification models and components for reproducing SOTA training results. Useful for more than just image classification, the library strives to make it easy to use the models as feature extractors for a wide variety of tasks (object detection, segmentation, etc).
The TPU Research Cloud (TRC) has been instrumental for improving training recipes and expanding the set of original timm trained model weights, especially for models that were developed from scratch without reference implementations. Having the ability to run numerous ablation experiments quickly is necessary to refine model architectures and training recipes to end up with robust, repeatable results.
Recent timm efforts that have relied on TRC include:
- A set of ImageNet-22k pre-trained -> ImageNet-1k fine-tuned model weights covering a range of popular CNN and ViT models
- A from scratch PyTorch reproduction of the MaxViT and CoAtNet hybrid ViT-CNN models (original model from Google Research)
- Research on integrating log-spaced relative-position bias (based on ideas in Swin Transfomer V2) into traditional Vision Transformer and CoAtNet/MaxViT architectures (successful weights available)
- Training recipe refinement for the paper ResNet strikes back: An improved training procedure in timm
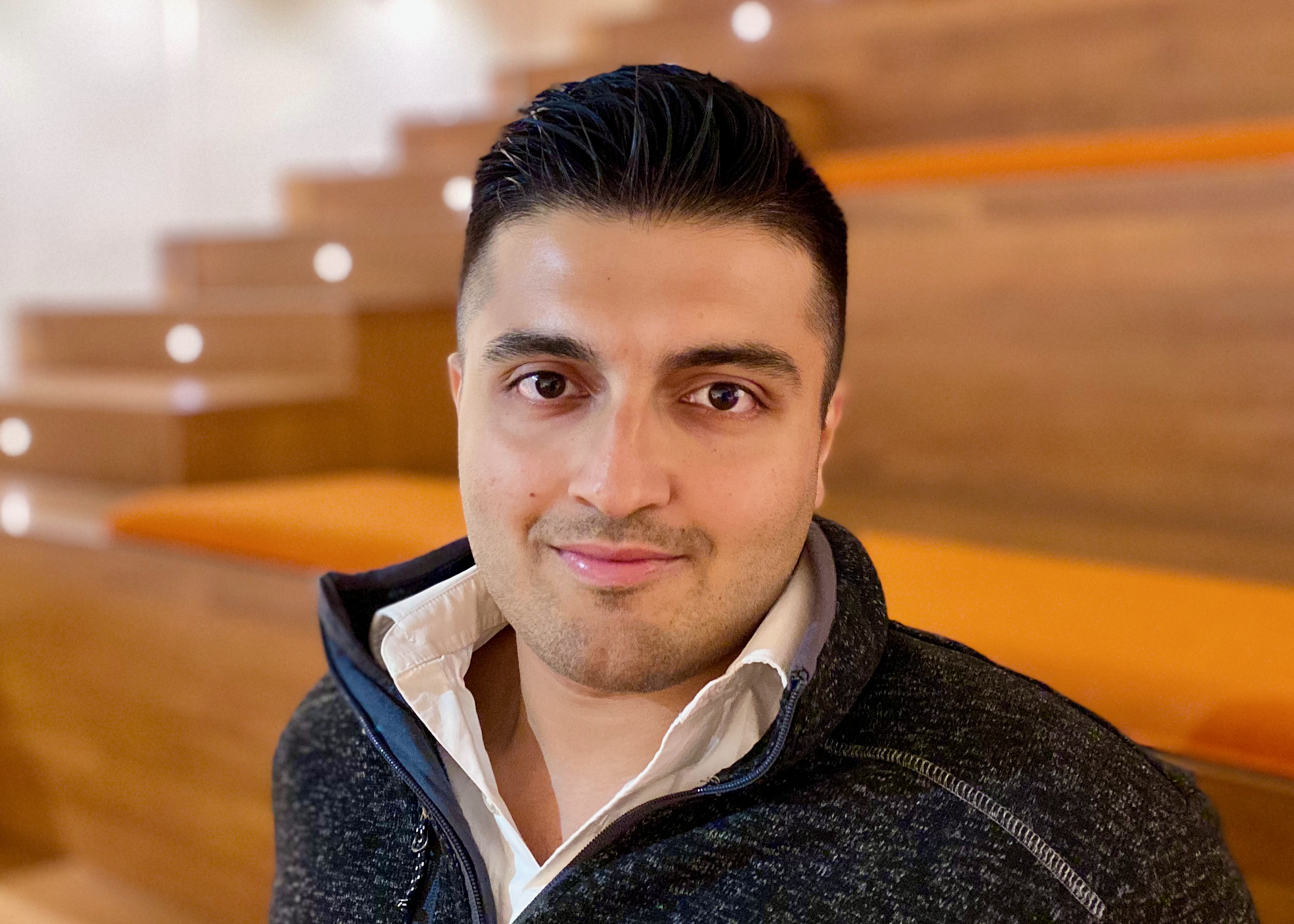
Eeshit Dhaval Vaishnav
I am Eeshit Dhaval Vaishnav and I am writing to express my gratitude to the Google TPU Research Cloud (TRC) program for their generosity.
I was a PhD student at MIT, advised by Prof. Aviv Regev, when we participated in the TRC program. The research conducted during our tenure in the TRC program was carried out in collaboration with Dr. Carl G. de Boer, in addition to Dr. Joshua Z. Levin's and Dr. Francisco A. Cubillos' research groups.
The research focussed on building a sequence→function framework, using gene regulatory DNA as the demonstrative example. We built this framework around an 'oracle' that we developed using a deep neural network model that predicts gene expression given a regulatory DNA sequence. This 'oracle' can be used to study the evolutionary history and future evolvability of DNA sequences for diagnostic applications, as well as to design DNA sequences for therapeutic, synthetic biology and biomanufacturing applications.
The paper based on this research was published on the cover of Nature, highlighted in Nature News and Views, and recognized by the broader scientific community.
I thank Google TRC for their continued support, and will make sure to pay it forward.
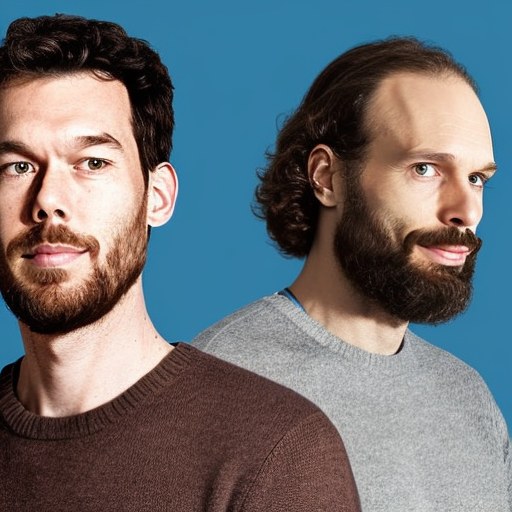
Martin Müller & Florian Laurent
Hello! We are Martin Müller and Florian Laurent, two Machine Learning researchers. Since the beginning of 2020, we've joined our efforts to work on generative AI research for both text and vision.
As text generation led to unprecedented results for the English language in 2021, capabilities in many other languages were still fairly weak. This is why we built Cedille.ai, a fine-tuned version of EleutherAI's GPT-J model, unlocking generative AI for the French language. As summarized in our recent paper, the model achieved competitive scores in various French language tasks (even beating GPT-3 on release!) and is available in open source.
In June of 2022, we set ourselves the goal to crack photorealism for image generation. We started our efforts by scaling up both data and model size for StyleGAN and diffusion models, adapting training code for TPU pods. The results are promising - check out our GitHub page for the latest code and models.
Both of these projects (as well as previous work with Per E Kummervold, see below) were made possible thanks to generous compute budgets provided by TRC as well as novel ways of doing large-scale model training in the JAX ecosystem.
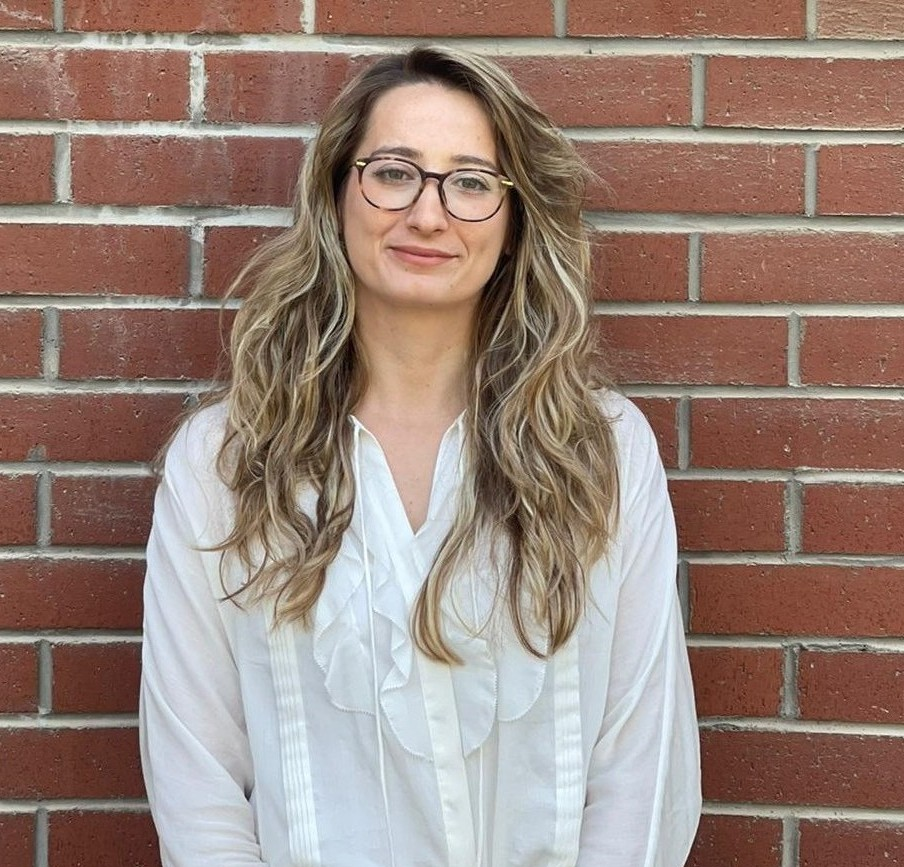
Hazal Türkmen
My name is Hazal Türkmen. I'm currently a Ph.D. candidate at Ege University, Turkey, working on clinical natural language processing advised by Oğuz Dikenelli. My primary interest is AI for healthcare, specifically developing large-scale language models in a low-resource language such as Turkish.
After the impressive performance of BERT in several downstream NLP tasks, using pre-trained language models became the standard engineering approach for NLP systems. However, training these models is highly costly and extremely limited to English. In our latest work, we developed the first publicly available biomedical BERT models in Turkish, BioBERTurk family, using the cutting-edge resources provided by the TCR program. For model evaluation, we also created a text classification task for head CT radiology reports in Turkish working with radiology experts from Ege University Hospital.
The most important support that the TRC program provides us is access to TPUs devices, which allow us to investigate various pre-training strategies using our biomedical and task-related corpus.
More recently, we have been working on different pretraining and fine-tuning approaches in clinical Turkish domains and evaluating our models for different tasks in clinical NLP like classifying mammogram reports according to the BIRADS standard.
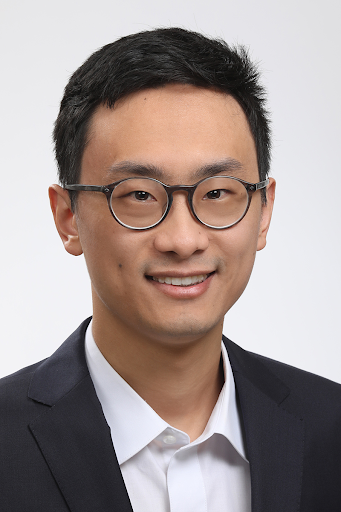
Yang Song
My name is Yang Song, and I'm a PhD student from Stanford, advised by Stefano Ermon. I work on deep generative models, as well as their applications to AI safety and inverse problem solving.
Although generative models are important for many applications in machine learning, they are quite challenging to train. Existing methods either have to use the notoriously unstable adversarial training procedure, rely on restricted model architectures, or require approximate loss functions for successful training. To overcome these challenges, we developed a new family of generative methods termed score-based generative models. Our approach overcomes the aforementioned challenges, achieving record-breaking performance in various generation tasks. The idea is widely used in applications including image generation, audio synthesis, shape generation, and plays an important role in recent high-profile projects such as OpenAI DALLE-2.
The generous support from TPU Research Cloud (TRC) has been absolutely critical for the development of score-based generative models. With TRC, we have 1) proposed a Stochastic Differential Equation formulation of score-based generative models; 2) demonstrated its connection to variational inference, and its ability to achieve superior likelihoods on real datasets; and 3) developed a successful application in solving challenging inverse problems in medical imaging.
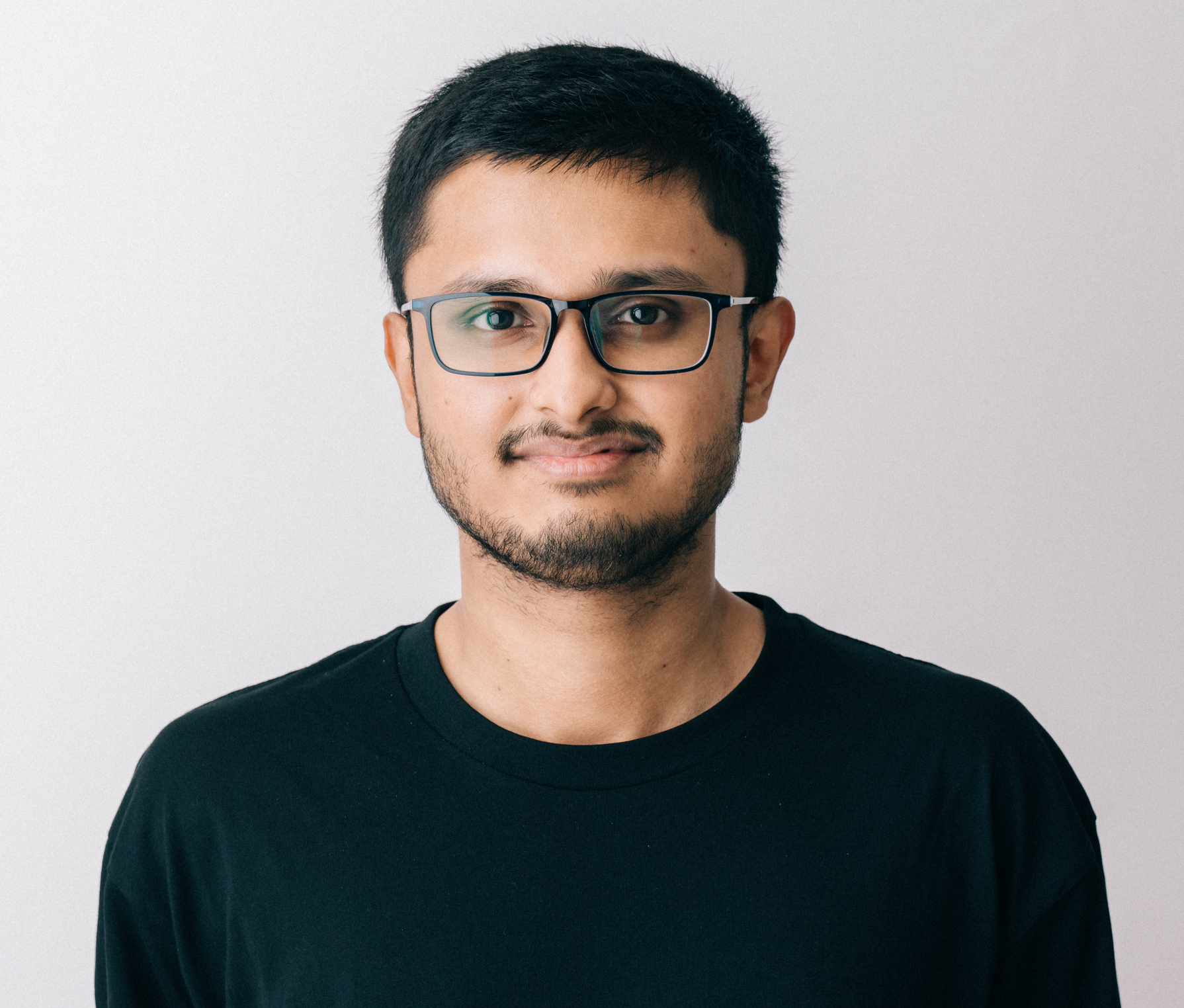
Sayak Paul
Aloha! My name is Sayak. I'm a Machine Learning Engineer at Carted focused on representation learning from webpages as well as NLP use cases. My personal interests are in computer vision (self-supervision, semi-supervision, model robustness, and so on). I joined the TRC program back in 2019 (when it was TFRC) and since then I've used its benefits.
In my early days, I used it to train a blood cell detection system for histopathologists. More recently, I used it for knowledge distillation-related experiments: this CVPR paper and a reproduction of this Google Brain paper. The latter work is particularly important because it allows practitioners to train state-of-the-art student models for efficient deployments. Last year, I used TPU v3-8s to evaluate the JAX implementations of Vision Transformers (ViT) on robustness benchmark datasets for a larger investigation. This work was accepted at AAAI-22. With a couple of friends, I developed an end-to-end colorization system in TensorFlow that is fully compatible with Cloud TPUs. Even though we never published that project, Cloud TPUs really helped us to iterate faster. For image generation tasks like colorization, speedy iterations are much needed.
I love to reproduce papers that I find to be interesting. For evaluating my implementations on benchmarks datasets like ImageNet-1k I often rely on TPU v3-8 pods. Currently, I'm tinkering with the big_vision codebase and experimenting with a few ideas of my own to improve the baseline ViT model it provides.
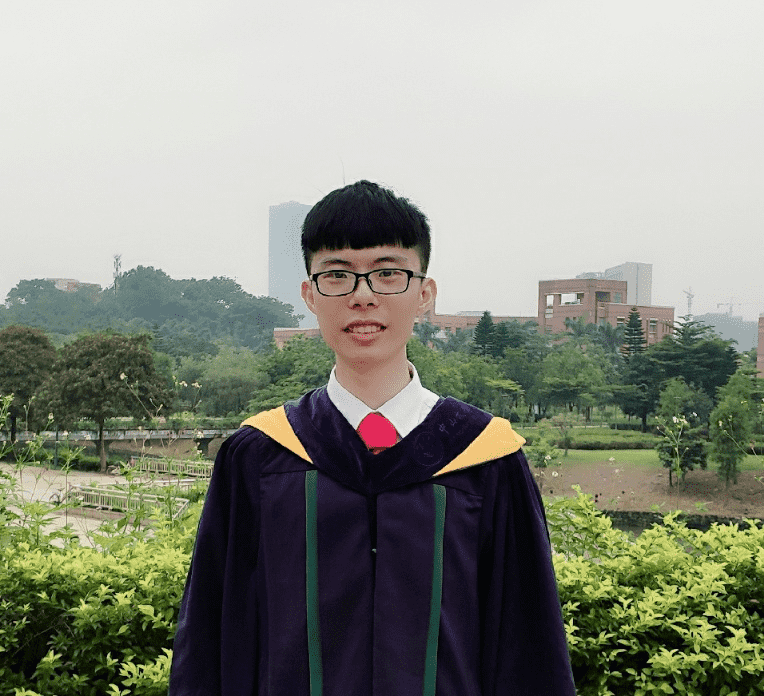
Weihao Yu
Hi, I am a PhD student at National University of Singapore (NUS). Thanks to the TRC program for the continued support. My research interest is deep learning, especially transformer models and their applications in computer vision and natural language processing.
In our ReClor paper (ICLR 2020), we introduce a logical reasoning dataset and find that current pre-trained transformer models have an outstanding ability to capture biases contained in the dataset but struggle in logical reasoning with poor performance, indicating more research is needed to essentially enhance the logical reasoning ability of pre-trained language models.
In ConvBERT (NeurIPS 2020 Spotlight) and LV-BERT (Findings of ACL 2021), we introduce convolution into pre-trained language transformer models to improve their effectiveness and efficiency.
In our MetaFormer paper (CVPR 2022 Oral), we abstract transformers into a general architecture MetaFormer and hypothesize that instead of specific token mixers, MetaFormer is more essential for the model to achieve competitive performance. To verify this, we replace the attention module in transformers with an embarrassingly simple pooling operator. Surprisingly, the derived model, PoolFormer, achieves competitive performance on multiple computer vision tasks, which well supports our claim.
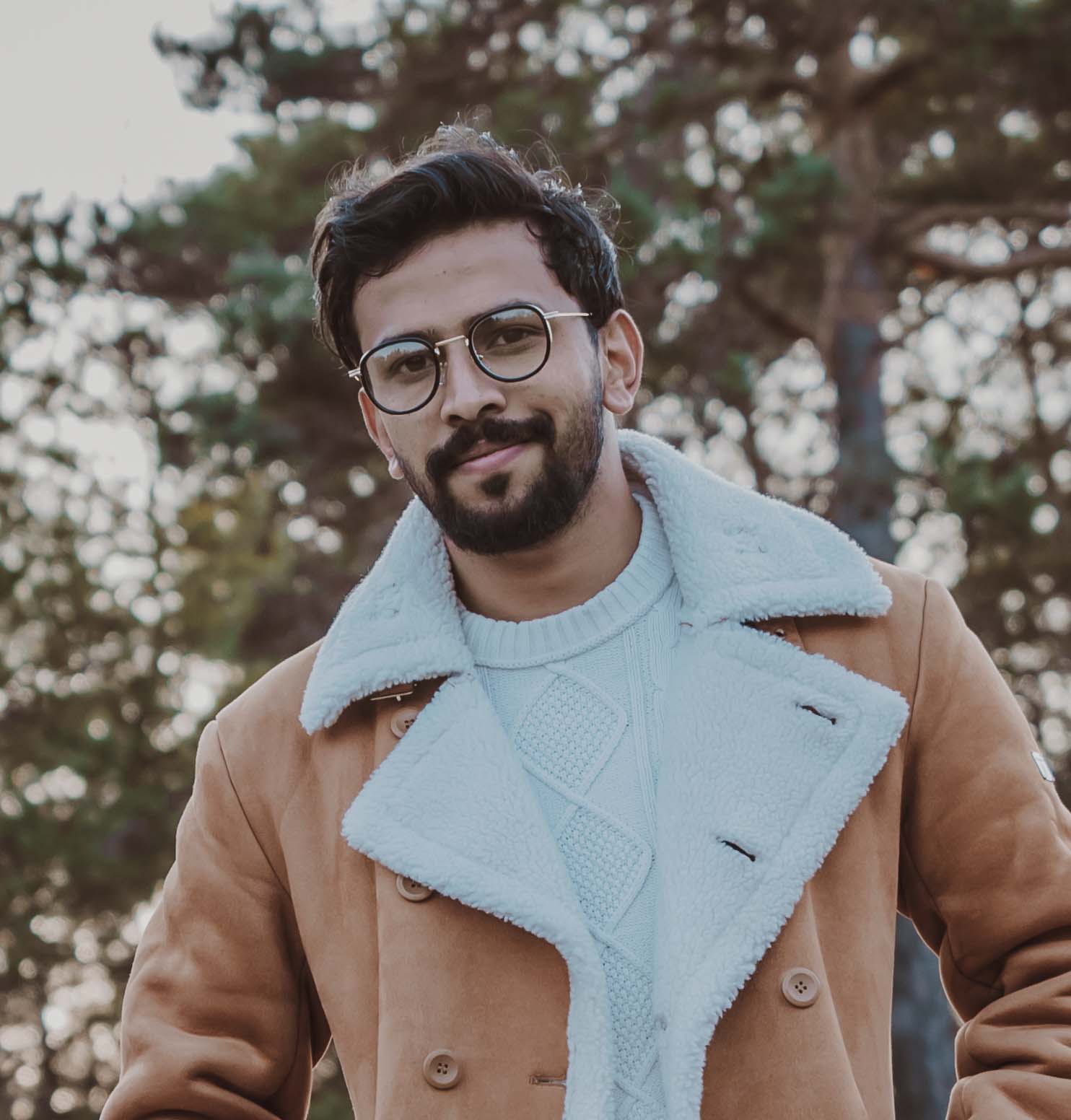
Kohulan Rajan
Dr. Kohulan Rajan is a young researcher from Sri Lanka. Currently working as a postdoctoral researcher in the Steinbeck Group at the University of Jena in Germany. He is primarily interested in cheminformatics, deep learning, and image recognition.
In scientific articles, a great deal of information has been published on chemical compounds, their structures, and their properties. However, only a fraction of this information is available in open databases. Retrospectively curating open data from books and journals automatically or semi-automatically, therefore, is a timely challenge[1]. Optical Chemical Structure Recognition (OCSR) tools are used to extract chemical structure depictions and convert them into computer-readable formats. DECIMER (Deep lEarning for Chemical ImagE Recognition)[2]an open-source automated software solution has been developed to address the OCSR problem through deep learning for image segmentation[3] and recognition[4]. STOUT (SMILES-to-IUPAC-name translator)[5] was developed to translate recognized structures into IUPAC names.
Kohulan is the lead author and developer of DECIMER and STOUT. They were trained primarily on cloud-based TPUs. DECIMER is now available to the public via decimer.ai.
[1] Rajan K, Brinkhaus HO, Zielesny A, Steinbeck C (2020) A review of optical chemical structure recognition tools. J Cheminform 12:60
[2] Rajan K, Zielesny A, Steinbeck C (2020) DECIMER: towards deep learning for chemical image recognition. J Cheminform 12:65
[3] Rajan K, Brinkhaus HO, Sorokina M, Zielesny A, Steinbeck C (2021) DECIMER-Segmentation: Automated extraction of chemical structure depictions from scientific literature. J Cheminform 13:20
[4] Rajan K, Zielesny A, Steinbeck C (2021) DECIMER 1.0: deep learning for chemical image recognition using transformers. J Cheminform 13:61
[5] Rajan K, Zielesny A, Steinbeck C (2020) STOUT: SMILES to IUPAC names using Neural Machine translation
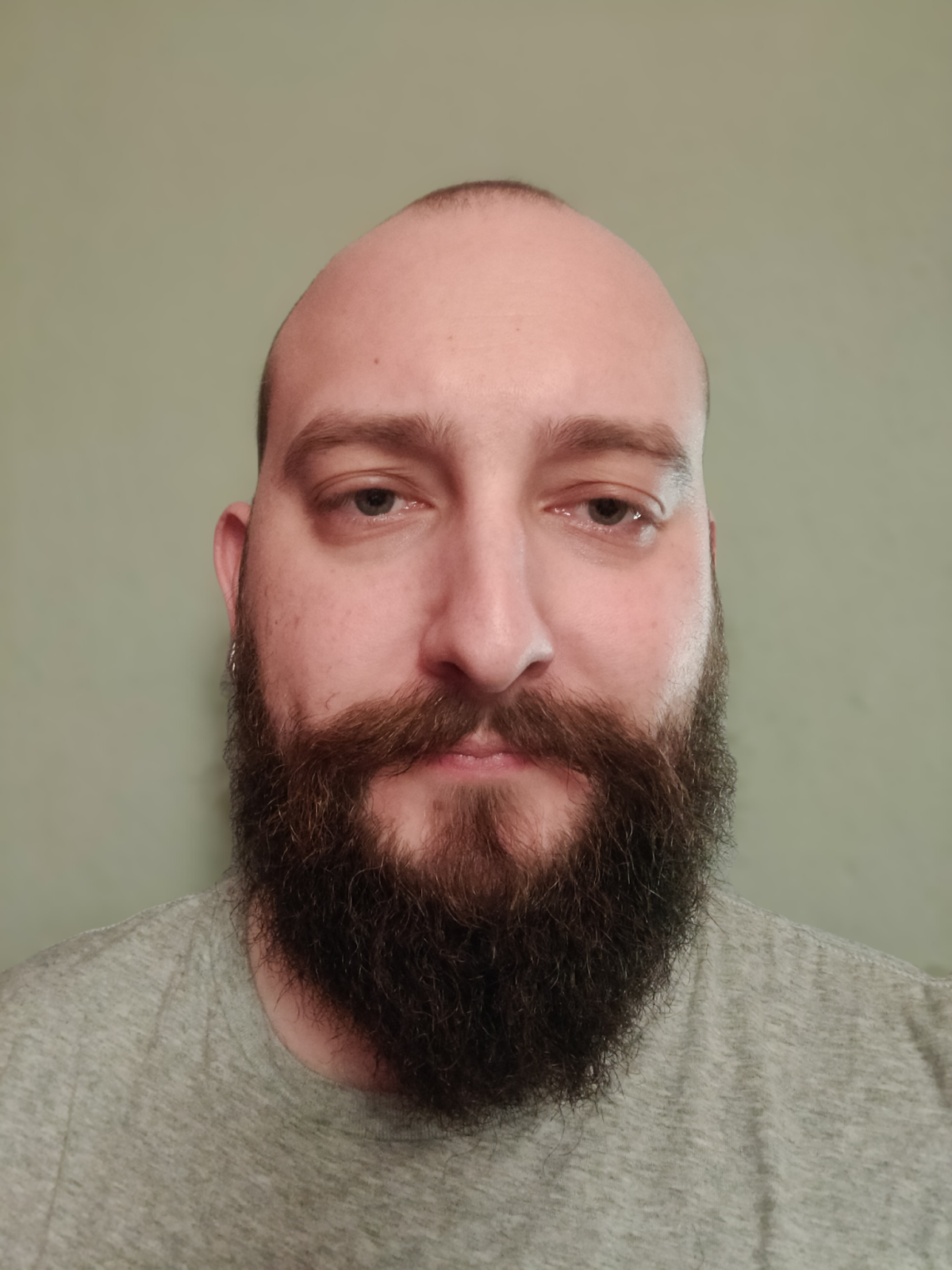
Nicholas Santavas
My name is Nicholas Santavas, and I am a PhD candidate with main interests around Machine Learning and Human-Computer Interaction, in the Laboratory of Robotics and Automation in Greece. I also work as a Research & Development engineer for a Dutch startup. One of my recent publications introduces a novel and very efficient Deep Learning architecture for Hand Pose estimation on images, combining Convolutional layers with a Self-attention mechanism, leading to an extremely lightweight architecture, resulting in just 1,9 M parameters network surpassing other state-of-the-art systems.
In my latest manuscript, we suggest an innovatory loss function that improves substantially the classification performance of a Neural Network, by enhancing intra-class compactness and inter-class separation. In this work, we focus on an effective hyperplane-based segregation which adds an extra penalty in the Softmax loss function depending on the network's discrimination capabilities between classes.
My work was greatly supported by Google's TPU Research Cloud program, providing me access to Google Cloud and TPU accelerators. I would like to express my appreciation for the backing of my research efforts.
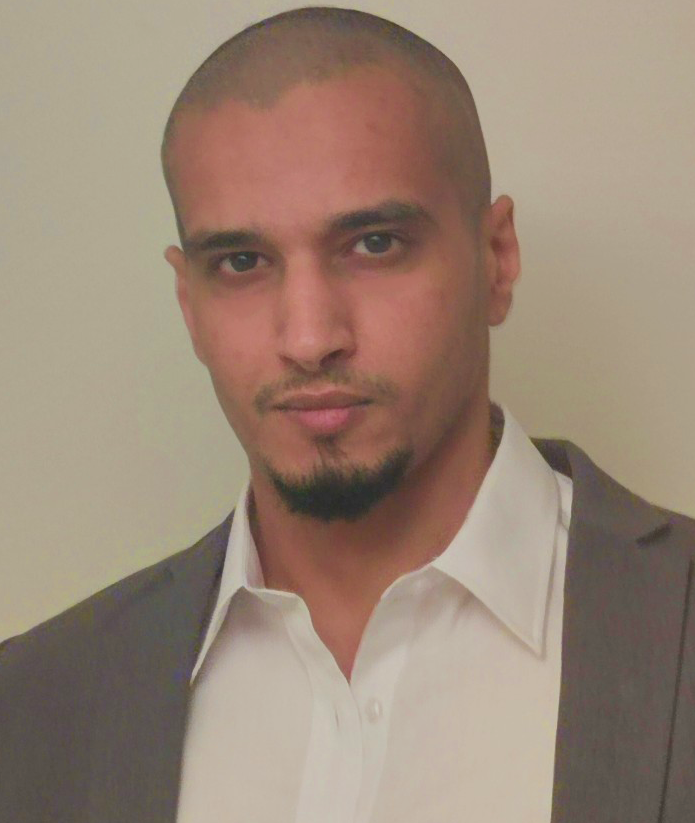
Sultan Alrowili
My name is Sultan Alrowili, and I am a Ph.D. student at the University of Delaware under the supervision of Prof. K. Vijay-Shanker, who has more than 30 years of research experience in the NLP area. Our research involves building a large biomedical language model (BioM-Transformers) based on state-of-the-art NLP models, including BERT, ELECTRA, and ALBERT. We trained our models on large biomedical corpora, including PubMed abstracts and PMC full articles. Our models serve various applications in the biomedical field, including Named Entity Recognition (NER) and biomedical text classification tasks such as ChemProt, GAD, DDI, and MedMNLI. We explain our large biomedical question answering models in our published paper.
Our models also are practical with biomedical question answering tasks such as BioASQ, COVID QA, and biomedical chatbots. We participated in the BioASQ9B challenge (2021) where we took the lead in two batches in List and Factoid questions. In 2022 we participated in the BioASQ10b challenge, where we took the lead in all four batches of List questions and three batches of both Factoid and Yes/No questions.
In addition to our research work in the BioNLP area, we have been actively working in the Arabic NLP domain where we built our efficient and State-of-the-Art Arabic language model (ArabicTransformer), which we published at EMNLP21.
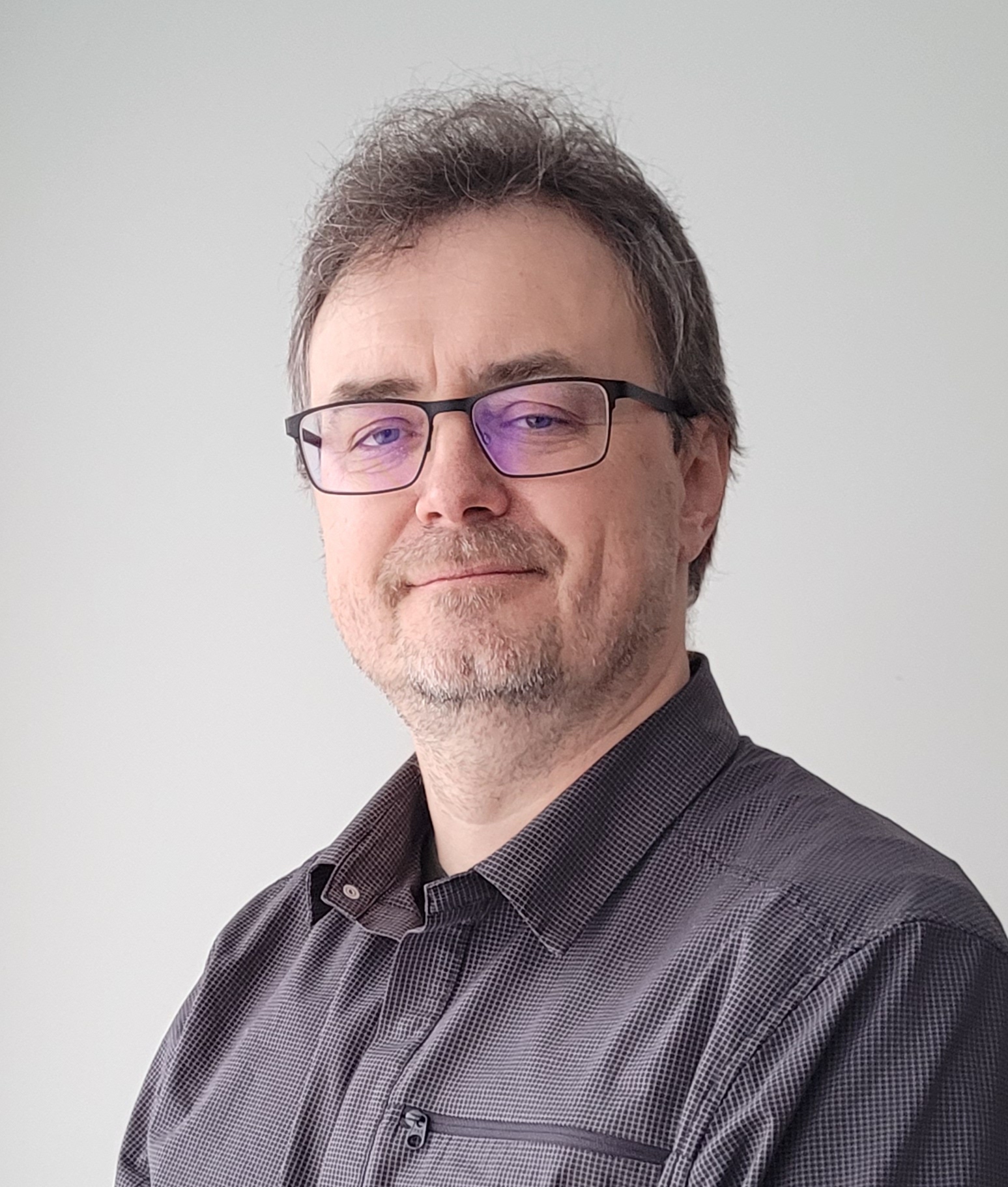
Per E Kummervold
I am a Norwegian researcher that is passionate about large language models and their implications. The resources from TRC have allowed me to do research experiments that would have been very hard without this support. I am together with the Vaccine Confidence Project at the London School of Hygiene & Tropical Medicine using natural language processing for getting a better understanding of vaccine sentiments.
While transformer-based models have yielded state-of-the-art performance in natural language processing, they still have had some problems with the specialised social media language. We were able to do domain-specific pretraining on a corpus of nearly 100M Twitter messages on the topic of COVID-19. Together with Martin Müller and Marcel Salathé from the Digital Epidemiology Lab at EPFL, Switzerland, we trained the COVID-Twitter-BERT (CT-BERT). The model gives a marginal performance increase of 10-30% compared to the base model, with improvements highest in evaluation sets that are closer to the target domain.
I have also been training Norwegian language models. Only five million people worldwide speak Norwegian, and the available textual sources found on the Internet are simply not sufficient for training well performing transformer models. The National Library of Norway (NLN) has positioned itself at the forefront of memory institutions globally by building an enormous collection of digitized materials. Together with Javier de La Rosa, Freddy Wetjen and Svein Arne Brygfjeld at the AiLab of the National Library the resources provided by TRC have enabled us to train robust transformer models for Norwegian with performance on par with English models.
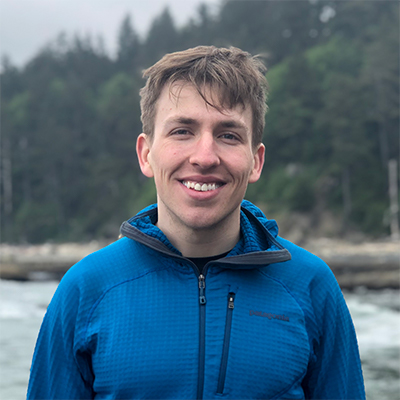
Rowan Zellers
I am a PhD student at the University of Washington advised by Yejin Choi and Ali Farhadi. My main research interest is multimodal commonsense reasoning: teaching machines to reason about "how the world works," both intuitively and in visual scenes, and express that through language. I've helped create several datasets that measure this, such as SWAG, HellaSWAG, and VCR. More recently, I've worked on two models for learning multimodal commonsense: PIGLeT and MERLOT.
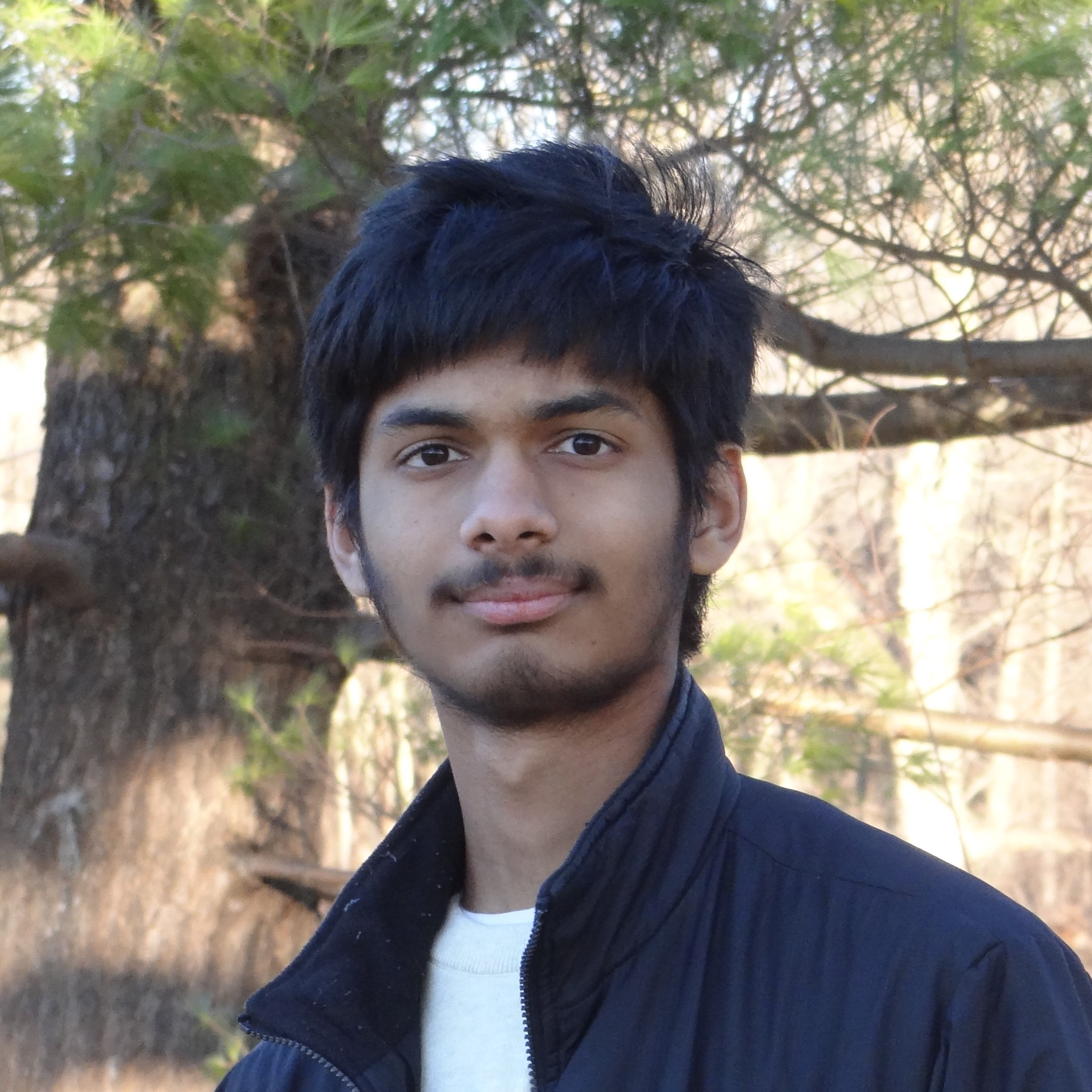
Arya Girish Manjaramkar
Hi! My name is Arya Girish Manjaramkar. I am a grade 10 student at North Park Secondary School, Brampton, Canada. A small team and I used Google's TPU Research Cloud (TRC) to fine-tune a large Transformer model (GPT-J) on Open-Source Code. GPT-J is a large six billion parameter model. Hence, it was not possible to handle it on traditional hardware.
The result of this experiment was CodeGenX. It is an Open-Source Visual Studio Code extension focused on Python Code Generation. We are now making it more accessible by making CodeGenX compatible with additional editors and IDEs.
We are currently conducting introductory sessions for students in urban and rural regions. We walk them through important topics in Machine Learning and how they can use CodeGenX to make learning more efficient. These students often do not have valuable resources at their disposal. We are sure that the concepts they learn through these sessions will enable them to understand cutting-edge technologies.
We envision that students will find CodeGenX of great benefit in their educational journey, it will equip them with the right tools to excel at their career prospects and enable them to become valuable contributors in society when they enter the real world.
More Information:
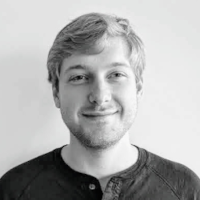
Andrew Yates
Andrew Yates is a Senior Researcher at the Max Planck Institute for Informatics, where he heads a research group at the intersection of Information Retrieval and Natural Language Processing, and teaches related courses at Saarland University. Yates is the lead author of the open-source Capreolus toolkit for building ad hoc retrieval pipelines [1], such as multi-stage pipelines that use an efficient method to rank candidate documents likely to be relevant to a given query before improving the ranking using a less-efficient neural method. Capreolus implements state-of-the-art neural ranking models and facilitates experiments on standard benchmarks. Thanks to the TPU Research Cloud's support, any Capreolus TensorFlow model can seamlessly be run on GPUs or on Cloud TPUs. One example is the PARADE model [2], which was developed using Cloud TPUs by Canjia Li while working in Yates' group. By considering the relationships among passages in a long document, PARADE achieves state-of-the-art effectiveness on several standard benchmarks while requiring substantially fewer parameters than similarly-effective approaches. This approach has also performed well on new tasks like the TREC-COVID Challenge. Moving forward, Yates' group is exploring how query and document representations can be enriched with information about key entities to further improve ranking.
[1] A. Yates, K. M. Jose, X. Zhang, and J. Lin. Flexible IR pipelines with Capreolus. In Proceedings of the 29th ACM International Conference on Information & Knowledge Management, pages 3181-3188, 2020.
[2] C. Li, A. Yates, S. MacAvaney, B. He, and Y. Sun. PARADE: Passage representation aggregation for document reranking. arXiv:2008.09093, 2020.
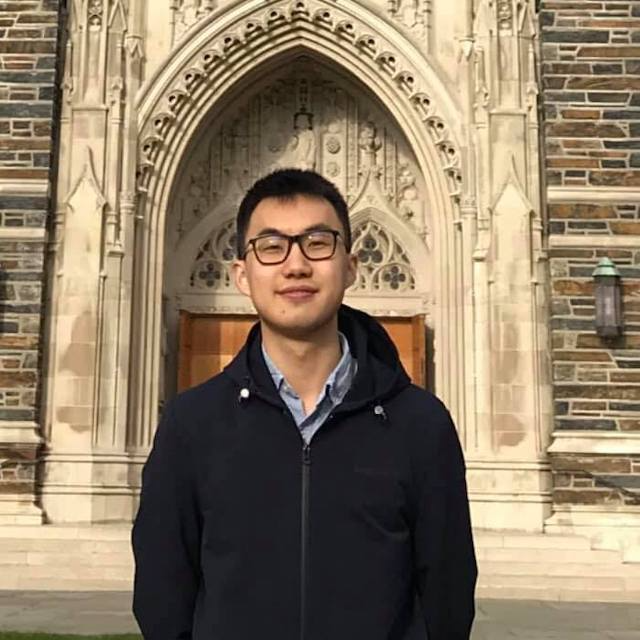
Ruiqi Zhong
My name is Ruiqi Zhong, a second-year EECS Ph.D. at UC Berkeley, working on Natural Language Processing.
The community has witnessed a trend that larger pre-trained language models lead to higher accuracy. However, are larger models better at every single datapoint, or is there any datapoint where larger models systematically fail on?
I investigated this question in my ACL 2021 paper. We first observe that it is challenging even to pinpoint datapoints where smaller models are better. The reason is that, even if we train the same-sized model twice with different random seeds, they might disagree on 8% of the datapoint, whereas BERT-Large improves the accuracy over BERT-Base by 2.5%. Therefore, we might observe smaller models to be better on some datapoint merely due to statistical noise.
To de-noise, we pre-train 10 times for each model size and fine-tune 5 times for each pre-trained model with different random seeds. This is extremely computationally heavy, but we managed to accomplish this with Google's TPUs. Along with the help of a new statistical method, we find that larger models are indeed worse on 1-4% of the datapoint across many datasets.
Are Larger Pretrained Language Models Uniformly Better? Comparing Performance at the Instance Level
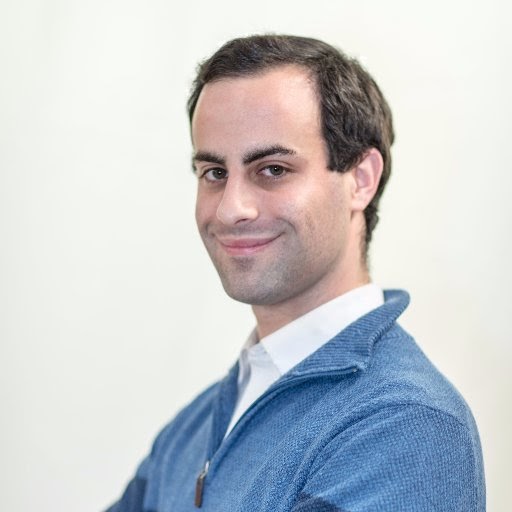
Jonathan Frankle
Over the past two years, the Programming Systems Group at MIT (led by Professor Michael Carbin) has used the TPU Research Cloud (TRC) as our primary research infrastructure for a number of projects related to neural network pruning and sparsity, most notably our work on the Lottery Ticket Hypothesis. The TRC made it possible for us to, among several other projects, explore our lottery ticket findings at much larger scales (Jonathan Frankle, Gintare Karolina Dziugaite, Daniel Roy, Michael Carbin, ICML 2020), develop state-of-the-art methods for fine-tuning pruned neural networks (Alex Renda, Jonathan Frankle, Michael Carbin, ICLR 2020 Oral), evaluate the state of research in pruning at initialization (Frankle, Dziugaite, Roy, Carbin, ICLR 2021), develop scaling laws to predict the performance of pruned neural networks (Jonathan Rosenfeld, Jonathan Frankle, Michael Carbin, Nir Shavit, ICML 2021), and further work to be released soon. Our style of research involves rigorous empirical analysis of deep learning phenomena, an approach that requires significant amounts of compute to ensure that findings are robust. The TRC has made it possible for us to run each experiment multiple times across a range of settings, allowing us to convincingly present our findings about the nature of neural network sparsity.
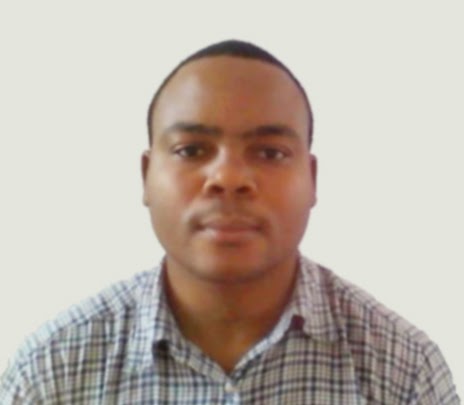
Ernesto Mboana
My name is Ernesto from Mozambique, I have a Mathematics Degree from Eduardo Mondlane University, a local University. I have been involved in web development and programming for most part of my professional career and I always looked for ways to conciliate it with my passion for mathematics research. The new dawn of Deep Learning and it's potential, offered such an opportunity, so I found myself reading more and more about it and taking some online courses. I have been particularly interested in Natural Language Processing and how I could integrate with my personal web related projects.
Eventually I found out about TensorFlow Research Cloud (TFRC) and applied. It was a huge opportunity to train and finetune some models and to put to test some ideas I had, eventually I managed to deploy them in some of my projects:
- QuantoSei: Focusing on offering AI tools for Education and rapid information retrieval, including exams simulation, autocomplete, summarization, question generation, question and answering features, mainly in Portuguese language.
- NoticiasAI: A local news aggregator website, that aims to distill and create insights from news, including news similarity, sentiment analysis, predominant actions, distillation, summarization and translation.
It has not been an easy journey, most times would not know how to proceed when facing a particular technical difficulty, but additional research and the web has always helped to move forward and master every new technique.
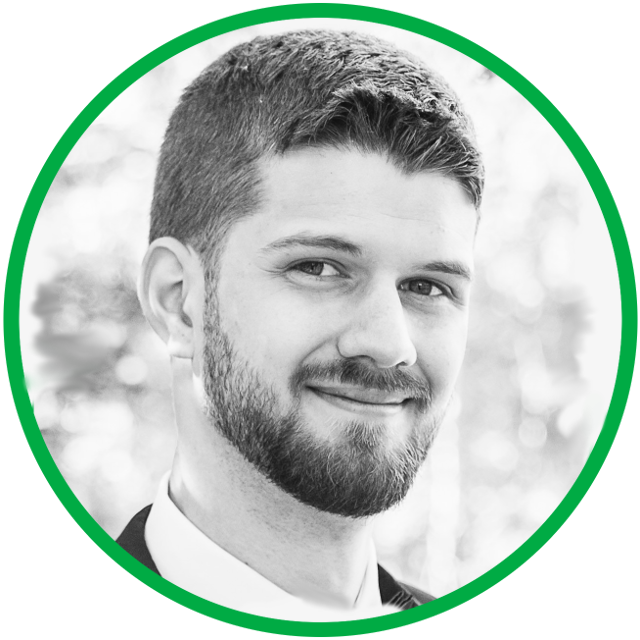
Evan Crothers
Evan Crothers is a Computer Science PhD student at the University of Ottawa, working under the supervision of Dr. Herna Viktor (University of Ottawa) and Dr. Nathalie Japkowicz (American University), where he focuses on applications of large neural network language models to improve trustworthiness of online social spaces.
Evan was previously employed full-time in the Canadian federal public service for 6 years, where he worked to safeguard Canada from violent extremism. He is the youngest-ever recipient of the “Director's Merit Award”, and has further been recognized for his contribution to the Security and Intelligence Threats to Election (SITE) Task Force as part of the G7 Rapid Response Mechanism (RRM), protecting G7 democracies from threats to elections.
Evan's academic research was published in the IEEE MLSP 2019 conference paper Towards the Ethical Detection of Online Influence Campaigns, and focuses on methods of reducing algorithmic bias against non-native English speakers in language models trained to detect foreign influence operations on social media. This work was continued in his Master's thesis, Ethical Detection of Online Influence Campaigns Using Transformer Language Models. TRC made these experiments possible, allowing the development of new ethical and effective methods for detecting online influence campaigns.
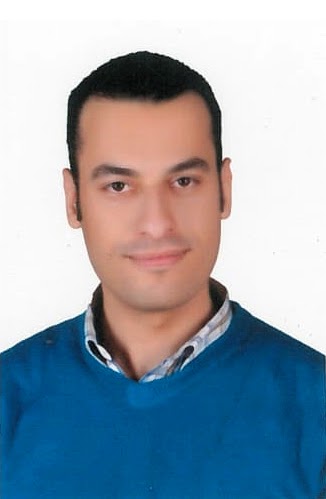
Ahmed Elnaggar
Ahmed Elnaggar is a research associate at the Technical University of Munich. His main focus of research is self-supervised learning on various modalities (Text, Protein, Source code, Images, and speech) using high-performance computing. TPU Research Cloud program allowed him to access Google TPUs, which provided enormous computing power to train deep learning language models (LMs). During training, these models utilized billions of unlabeled data, and during inference, they provided an accurate feature representation at low inference costs. Two of his recent breakthroughs are ProtTrans and CodeTrans.
In ProtTrans research, he trained six LMs up to 11 billion parameters on un-labeled data up to up to 393 billion amino acids. These models captured various biophysical features of protein sequences, which for the first time outperformed the state-of-the-art without using evolutionary information for tasks such as secondary structure prediction, thereby bypassing expensive database searches.
In CodeTrans research, he trained various encoder-decoder transformer LMs up to 0.7 billion parameters on 38 million un-labeled source code projects for nine programming languages (Python, Java, Go, Php, Ruby, Javascript, C#, SQL, Lisp) and one human language (English). The fine-tuned models outperformed the state-of-the-art models on thirteen software engineering tasks, including code generation and code documentation generation.
Papers
GitHub repositories
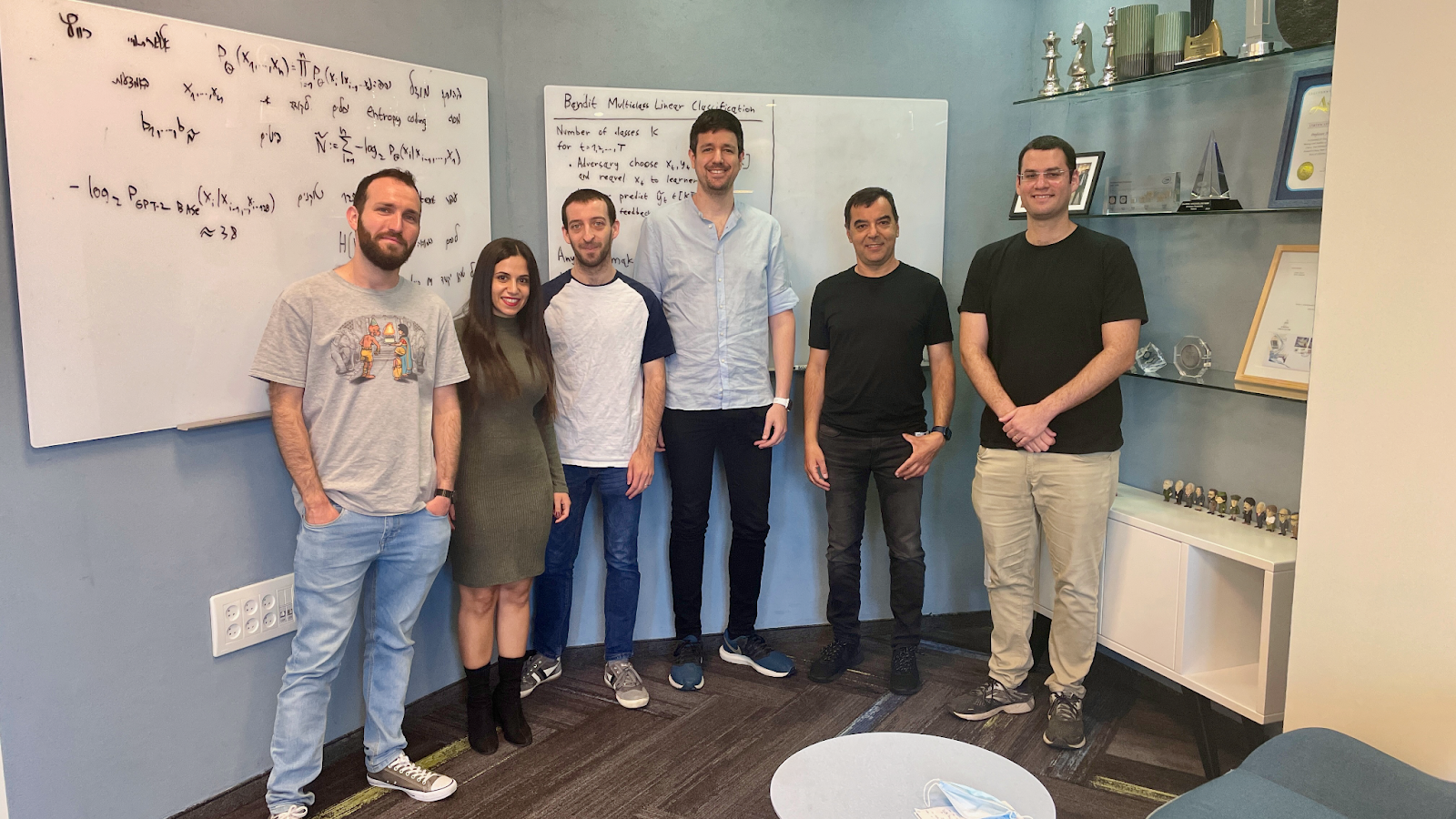
Prof. Amnon Shashua's Lab
In our lab, led by Prof. Amnon Shashua with graduate students Or Sharir, Yoav Levine, Noam Wies, Hofit Bata, and Daniel Jannai, we theoretically investigate the mechanisms behind prominent deep learning techniques, and leverage these insights to drive practical innovations.
Lately, we focus on self-attention networks (aka, Transformers), which facilitated recent breakthroughs in language understanding, and are showing promising signals in various other domains.
Our unexpected theoretical findings below were empirically reinforced by targeted and comprehensive experiments (100s of trained models), facilitated by the TFRC program computational resources.
The depth-to-width interplay
(NeurIPS 2020):
Depth has long been suggested as Deep Learning's source of success. However, we prove
that in self-attention networks the power of depth can only be unlocked when the width
of the model is above a certain threshold. Our results point to inefficiencies in
commonly used architectures and prescribe a simple practical formula for the optimal
depth-width ratio per parameter budget.
Which Transformer architecture fits my
data? (ICML 2021):
Just as depth is limited by width, we prove that width itself is limited by the rank
of the input vocabulary matrix. This bears special implications for cutting edge
efforts for utilizing self-attention in non-language domains (e.g., images).
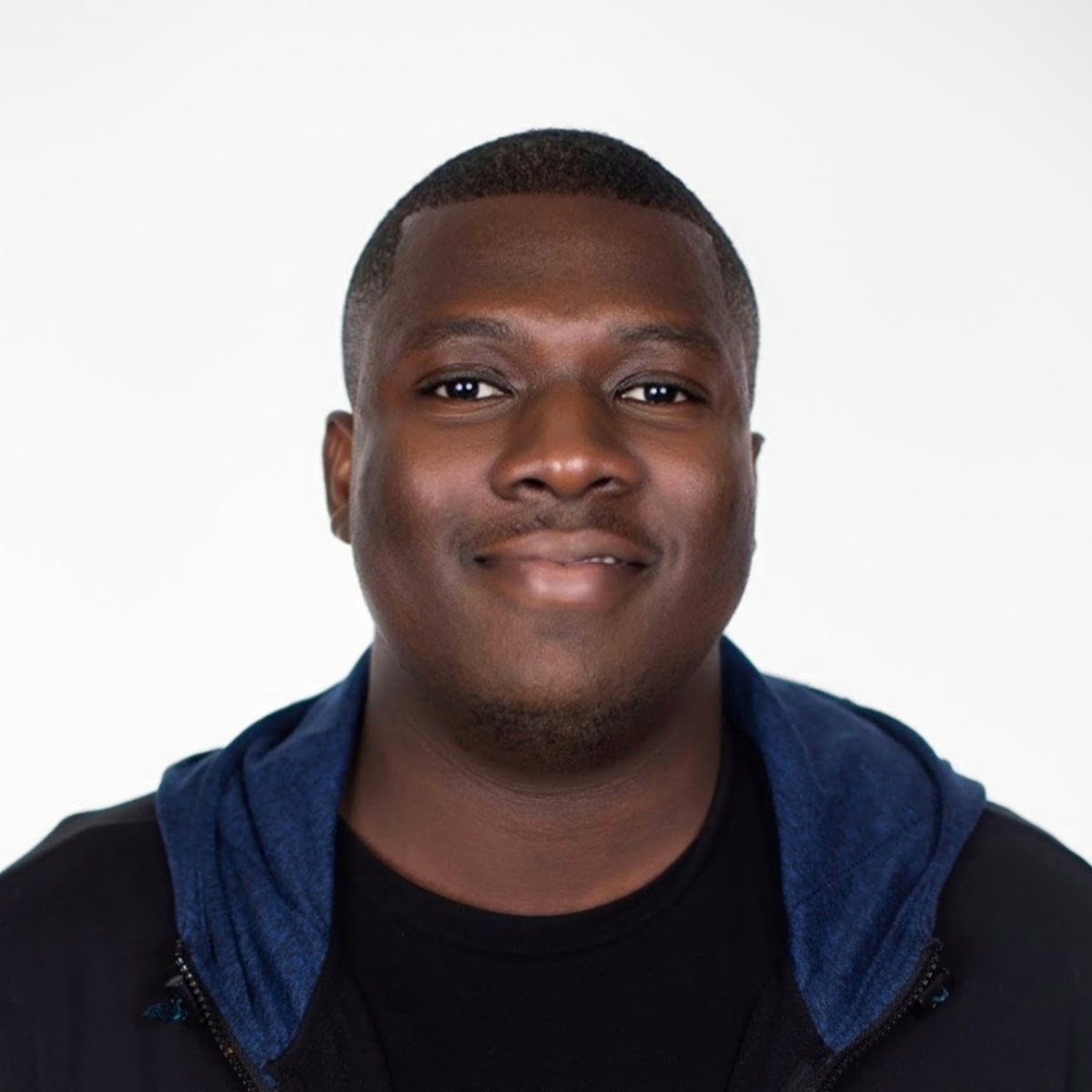
Wisdom d'Almeida
Hi! I'm Wisdom and I joined the TRC program in 2018 when it was still TFRC :) Access to the TRC Compute helped me run large-scale experiments on radiology report generation from Chest X-ray images. The idea was to train powerful language models on image and text data jointly, in such a way that models can generate clinically-pertinent natural language reports (including findings and impression sections) at test time, in order to support Chest X-ray disease predictions with some textual evidence or explanation. The output of my work constituted the main portion of my Master's thesis (not online unfortunately), and motivated further research on imbuing clinical awareness into language models with design and data inductive biases. Some of these follow-up works, still backed up by TRC Compute, were presented at venues such as Stanford's Frontier of Assisted Care Scientific Symposium, and the Montreal AI Symposium.