Text, images, code, tables or visualizations should have a reason to be presented in their entirety, to help readers justify their decisions without entirely shifting responsibility onto them.
The Data Card should always be an accurate and truthful representation of the dataset that advances the reader’s mental model of the dataset and its implications in practice. This is critical to building long-term trust and accountability with readers of Data Cards.
Provide readers with facts that can persuade and dissuade readers in their decisions.
This reduces selection bias, unintended misdirections or omissions that can lead to preventable misuses. However, note that this is not the same as providing contradictory information, which will simply reduce the credibility of your Data Card.
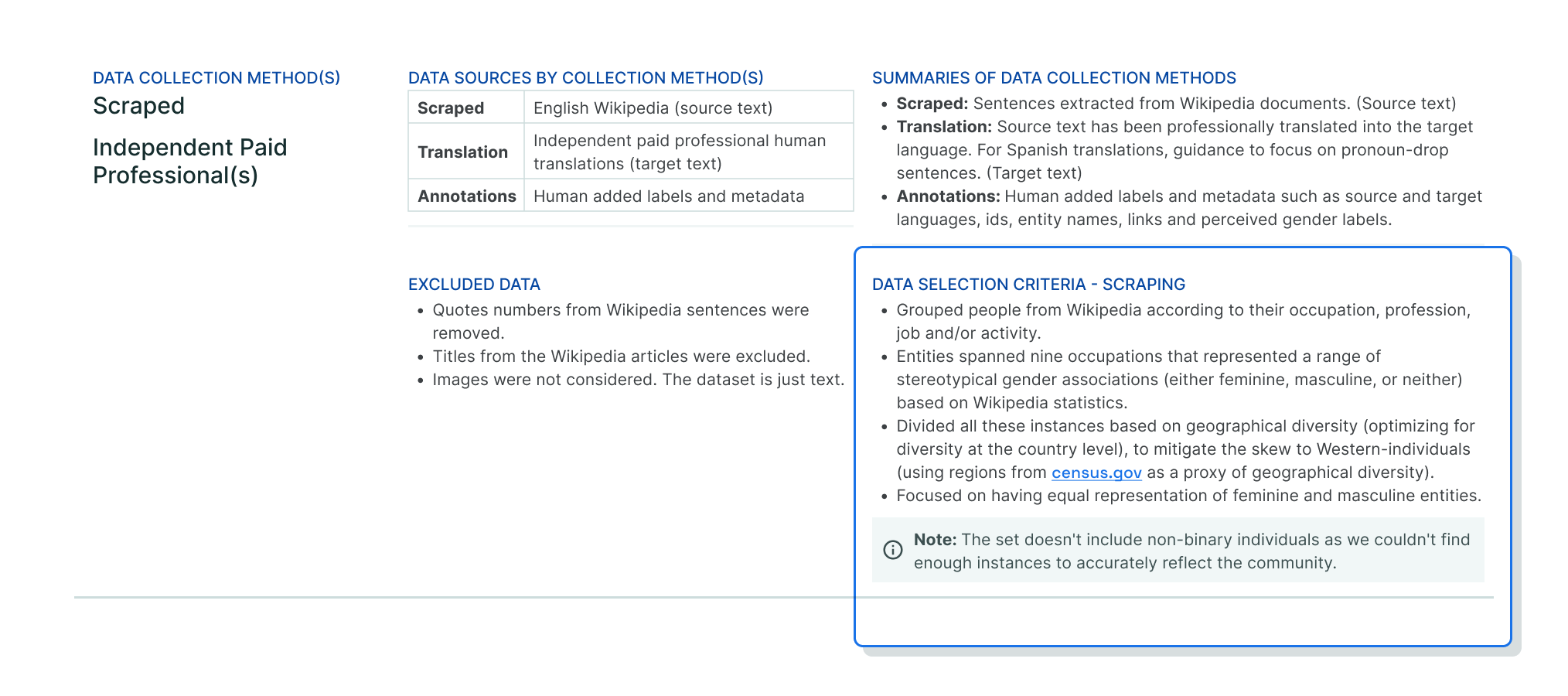
Information framed at unsuitable fidelities or without the right context forces readers to make assumptions about the dataset.
Provide sufficient context and use language that is suitable for your reader’s proficiency levels. This could look like annotating examples of datapoints with clear descriptions, or providing descriptions with increasing amounts of detail for more advanced users.
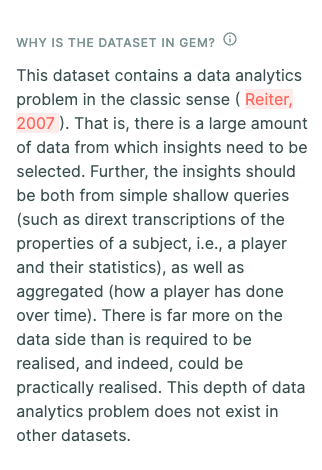
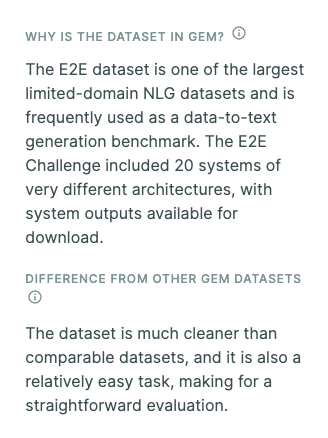