Fairness and bias looks different for different datasets, so determining fairness goals for your dataset and including them in your Data Card is an important driver for informed decision making. Provide a well-rounded explanation for fairness in your dataset.
Some biases can’t be mitigated, some are desirable in that they reflect real world behaviors. Even if there are no solutions or workarounds, inform readers so they can factor in this information when making decisions about when and how to use your dataset.
Ground fairness in real world contexts.
Fairness criteria and thresholds that are unconnected to the practical applications of the dataset or model can feel arbitrary. Three things you can do to operationalize the fairness goals of your dataset are:
- Consider your primary use case and set fairness criteria that reflect adversarial product tests that are similar to yours.
- Consider the limitations of your dataset and set fairness criteria that directly reflect the failure modes of your system.
- Consider the most important or valuable features of your dataset and set fairness criteria that describe the outlier utilities of more valuable or important features in your dataset.
Avoid pushing vulnerabilities under the rug.
Your fairness analyses can reveal inadvertent limitations in your dataset that may not be directly related to your intended use case. A proactive remediation is a healthy way to frame and prevent known risks in contexts beyond the scope of your motivations.
- Use your findings from your fairness analysis to articulate unsuitable or unintended use cases, therefore connecting them to real-world outcomes.
- Where possible, link to notebooks and visualizations to show your findings from fairness analyses!
- Clearly list recommendations for the future that dataset consumers can use to address limitations.
Example
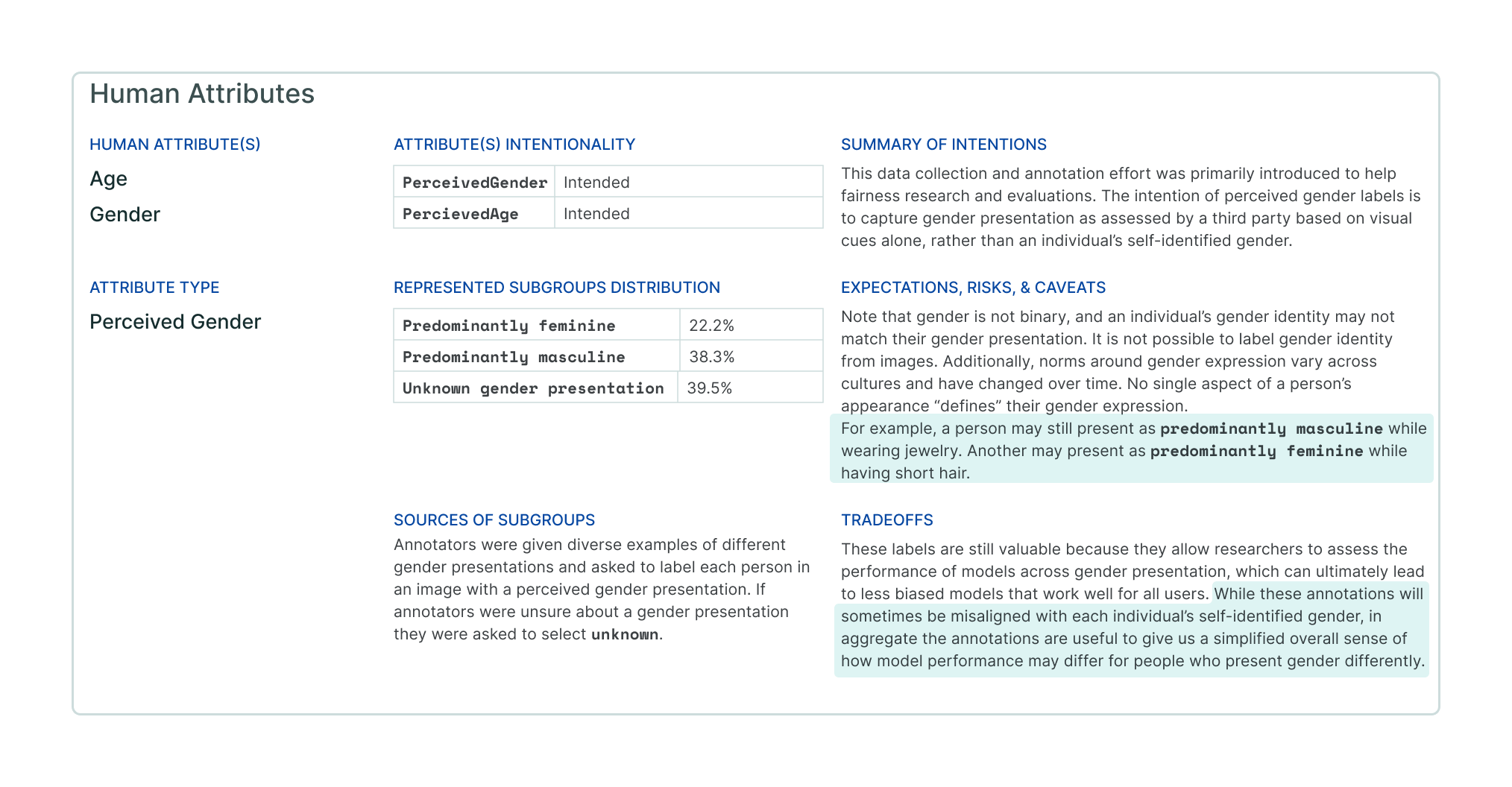