Some questions in a Data Card will require more effort and thought than others, which can impact your approach to standardizing or automating answers.
Some fields will be easier to standardize, such as a choice between different data modalities that can be used to describe multiple datasets; or information about upstream data sources that are unlikely to change across datasets. Similarly, fields that require repeated work to maintain recency and accuracy are easier to automate. Make sure you’re standardizing and automating the right things.
Automate and standardize responses that are less likely to change over time and across contexts.
Transparency artifacts document certain immutable facts and information that are resilient to upstream and downstream changes. Many times, this information is unstructured and cannot be learned from the dataset itself, though it always remains true and when absent, can easily introduce assumptions.
Automate and standardize both structured and unstructured information that rarely changes and remains relevant across a variety of contexts to ensure accuracy.
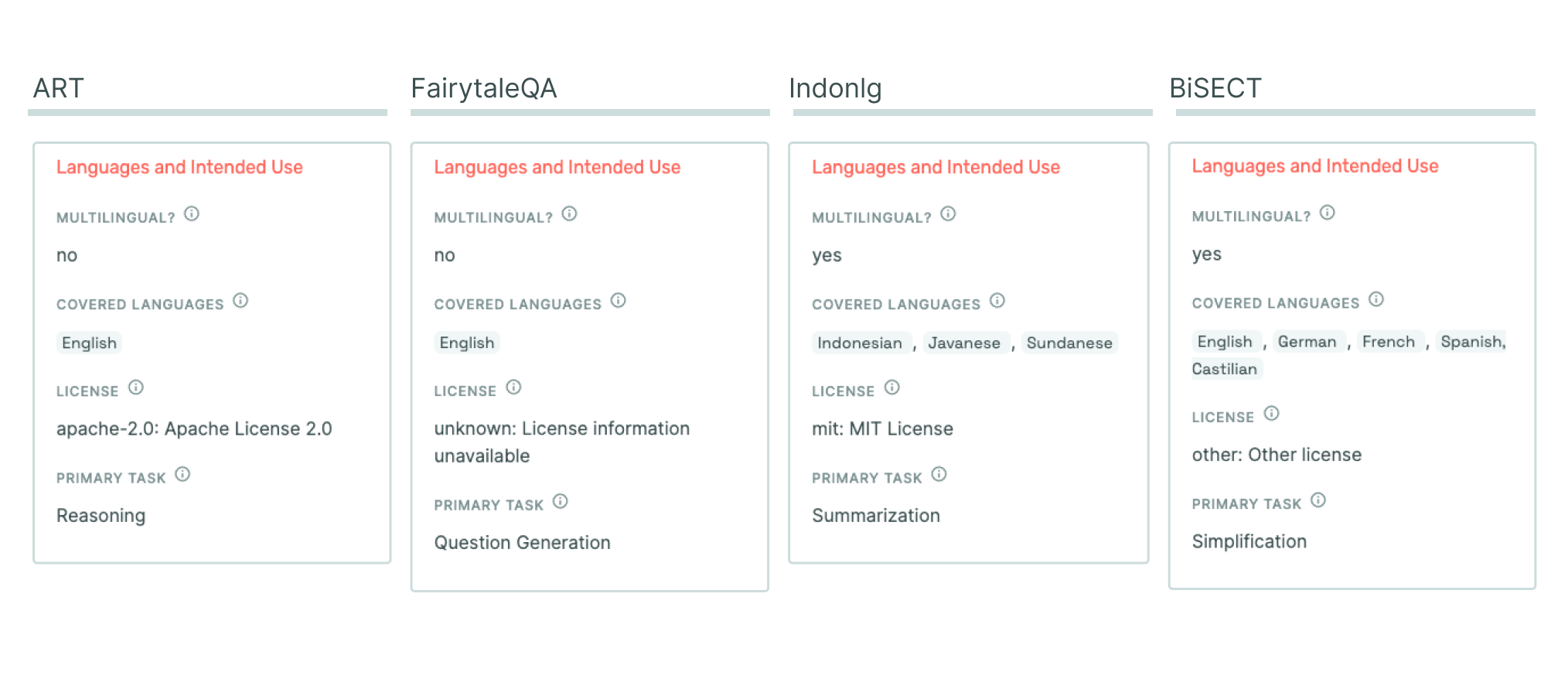
In certain contexts, automating answers can hide attributions necessary for trust.
Some answers require human accountability to be trusted, such as those about labeling policies or outcomes for marginalized communities. These are characterized by additional explanations or evidence, and should necessarily be traced back to a person.
Avoid automating these answers entirely, even if the processes used to arrive at results utilize automated tooling. Instead, automate answers that require inspectable calculation and/or algorithms to be trusted, such as an analysis that uses a Python package for floating point computation.
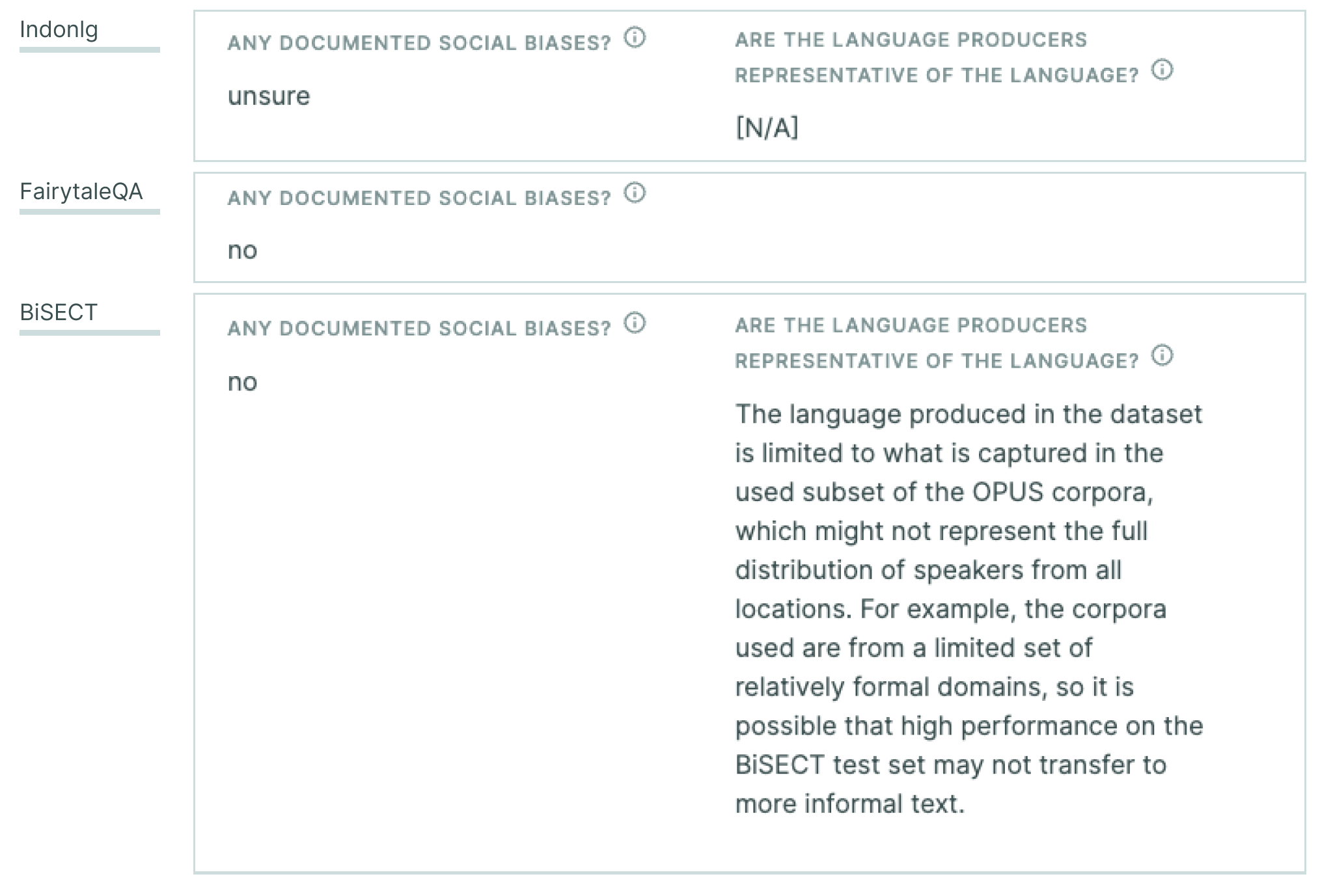